How Abacus Medicine Built a Modern Data and AI Stack with Databricks and Monte Carlo
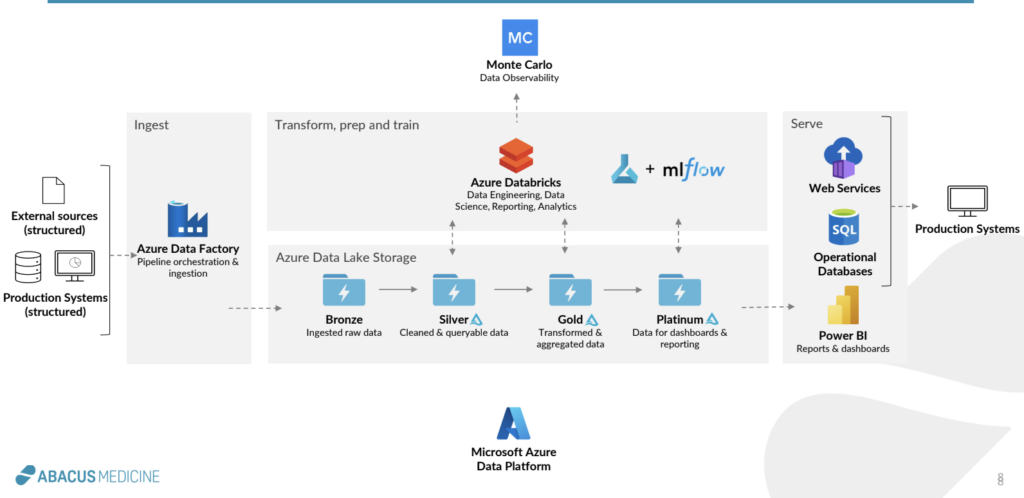
Copenhagen-based Abacus Medicine is working to make medicine more affordable and accessible for patients while saving costs for healthcare systems. The global pharmaceutical company buys and sells prescription pharmaceuticals across borders throughout the European Union — which involves complex data sourcing, handling, and quality assurance. In addition to the trading activities, Abacus provides pharmaceutical services helping in particular smaller and mid-sized companies commercialise their products in Europe.
The Abacus data team ingests and analyzes information on pharmaceutical availability, demand, and pricing across more than 20 procurement countries and 14 sales markets. And up until the last few years, that all happened within an on-premises architecture.
Fast forward to today: the Abacus data platform is 100% in the cloud, leverages Databricks for business critical machine learning applications, and supports a growing business that’s doubled its headcount and tripled its revenue since 2018. Safe to say their data team has been on quite the journey over the last five years.
So we’ve gathered some insights from Abacus’s Head of Business Analytics Malte Olsen and Data Engineering Team Lead Mariana Alves Monteiro, who recently shared a look behind the scenes at the modernization of their data stack — including how data observability plays a vital role.
Data challenges at Abacus
As a healthcare organization that serves multiple countries across Europe, Abacus ingests a lot of data — primarily through a fragmented assortment of batch jobs. “We have quite a fragmented data landscape, where various data sources and formats coexist, ” says Malte. “Within this complex landscape, we serve the needs of both operational and analytical functions using these diverse data assets.”
Abacus business users rely on timely, accurate data to make decisions around making trades, setting prices, securing new sourcing opportunities, and optimizing the supply chain. And when data isn’t fresh or complete, that decision-making is compromised.
Little visibility into legacy architecture
But, in the late 2010s, Abacus’s legacy, on-premises architecture was unstable and could not support advanced analytics scenarios. The data engineering team had little visibility into data health, and was experiencing significant problems around accessibility, flexibility, scalability and storage.
“We were aware that if a business user identified a data quality issue in a downstream report before it came to the attention of the data engineering team, it signaled a problem,” says Mariana.
As the team began to look at modernizing their data stack and migrating to the cloud, finding data quality issues at the source was going to become a major focus.
Lack of scalability in data testing
The data team were working systematically and rigorously setting up data pipelines, while looking for a more scalable way to ensure data quality to meet their growing data needs.
“The industry standards, up to this point, have been to enforce data quality by conducting rigorous testing of data assets,” says Mariana. “But we felt that going all-in on data quality testing was not scalable. It can be very tedious and time-consuming to create, update, and deprecate all the tests and cover our growing data state with a lot of quality checks.”
Finding a scalable solution to data quality was a top priority for Abacus’s data modernization efforts.
Lagging incident detection and resolution
Within their fragmented data landscape, quality incidents were cumbersome to handle. “Time to detection for data quality incidents could take days or weeks,” says Mariana. “And downstream users were often the first to notice when data was wrong.”
Once the data team became aware of an issue, reaching a resolution was just as time-consuming. They had to devote extensive resources to troubleshooting data incidents, diverting attention away from innovative projects and other initiatives that would improve their products and drive meaningful business results.
It was time for a change — a big one.
Data solutions at Abacus
In late 2019, the data team decided to invest in a cloud platform to enable data engineering, ML modeling, and BI services. The team spent nearly two years on their migration from a legacy system to a 100% cloud-based solution. It’s important to mention the organizational efforts that are required to succeed with such a migration and implementation of a modern data-stack. All units in the data team, like Business Intelligence experts, Data Analytics inhouse consultants, Data Scientists and Data Engineers were crucial to achieve this goal. One of the big enablers for such a transformation!
Migrating 100% of data to the Databricks Lakehouse
Today, the Abacus data team uses a modern stack built on Databricks, including Azure Data Factory for ingestion and orchestration, Databricks for transformation, Azure ML to deploy machine learning models, MLflow to populate web services with live models, Monte Carlo for data observability (more on that in a minute), and PowerBI for reporting.
Enabling self-service analytics at scale
The team set up a medallion architecture within Databricks to enable self-serve analytics at scale. Within their lakehouse, multiple layers of data are available to different teams for different business applications. The bronze layer is the “landing zone” of raw data. The silver layer is cleansed and queryable by multiple data teams. The gold layer contains reusable business data models, and the platinum layer is used for specific business use cases and reporting.
Investing in data observability with Monte Carlo
As the team began to migrate to the cloud, they knew they had to tackle their data quality problems and find a solution that could detect and surface issues as close to the source as possible. They began to learn about data observability — an approach that uses automated monitoring, root cause analysis, and lineage to improve end-to-end data health — and decided it was the right approach.
The data team looked into the available options and determined that Monte Carlo was the strongest solution on the market. But that still left one question: build or buy?
Ultimately, compared to the overall requirements of building their own solution — labor costs, opportunity costs, platform costs, compute costs — Monte Carlo was “definitely a much more cost-effective solution for us,” says Mariana. “We lacked the in-house resources to develop a data observability platform from scratch. We explored practical solutions that our team could construct, but many valuable features, such as machine learning models, were beyond the project’s scope.”
So the Abacus team implemented Monte Carlo, leveraging deep integrations with Databricks. Automated monitoring and alerting send notifications to specific teams when data falls outside historic patterns or custom thresholds.
The data team’s migration to the cloud — and to a modern data stack, including data observability — was successful.
Data outcomes at Abacus
A data team’s work is never done, but today, Abacus is reaping the benefits of its investment.
More scalable and reliable self-serve analytics
Together, the medallion architecture within Databricks and the end-to-end observability provided by Monte Carlo ensure that Abacus data consumers can access accurate, fresh, reliable data for their analytics needs.
When data pipelines break, the data team is now the first to know. This information allows them to communicate proactively with any downstream data consumers who may otherwise rely on impacted assets.
“The fact that we can get automated anomaly detection to identify data quality incidents before end users is a big outcome for us,” says Mariana. “If data is late, we can immediately notify stakeholders and deliver insights on the incident so that our stakeholders are not looking at stale data.”
Less time spent on data reliability
Instead of retroactively chasing fire drills and spending days on troubleshooting incidents, the data team now has more time to spend on productive work that adds value to the business.
“We’ve really seen the ratio of data assets to data engineers is decreasing,” says Mariana. “We have a decrease in time used for data profiling, the ability to deliver data asset insights to our stakeholders, and seen a decrease in our overall data downtime.”
Immediate time to value for data quality
When the data team was evaluating whether to build or buy their data observability solution, the time to value was a huge consideration. And Monte Carlo delivered.
“The expected time-to-value is months for building your own, versus two to four weeks with buying a solution like Monte Carlo,” says Mariana.
Rather than spending months of their team’s precious resources on building a custom solution, they were able to set up Monte Carlo within days. Since the platform’s models analyze historical data patterns to automatically set initial thresholds, the team started receiving notifications — and seeing improvements to data quality — almost immediately.
The future of data at Abacus Medicine
While their major migration project is complete, there’s still work to be done to improve data quality and drive even more business value from data.
The team plans to set up Unity Catalog in Databricks. “We want to leverage lineage and get that lineage into Monte Carlo,” says Mariana, “to democratize access to lineage data and directly impact data downtime by reducing the time-to-resolution.”
They also plan to improve how they track and measure those metrics, Mariana says. “We’re really working on how we can define and measure our KPIs, like data downtime, time-to-detection, and time-to-resolution of quality incidents across our data stack.”
The data team also wants to democratize data ownership across the organization, getting more business users involved in data incident resolution. “We have an ambition to have a system that covers all of our data sources as generalizable when we get a new data source,” says Malte. “This would cover a broad spectrum of our data landscape, giving insights to the user and getting input from them. We want to increase their data literacy by looking at the dashboards in Monte Carlo and figuring out what’s going on with the data.”
And, like nearly all data-driven businesses, the Abacus team plans to use generative AI to improve their workflows and support internal customers. As they work to make more data accessible to more consumers, data quality will continue to be essential — and data observability will continue to be a piece of the Abacus data stack.
If your team needs to improve reliability in the cloud, Monte Carlo is here to help. Contact our team to learn more about how data observability can improve freshness, accuracy, and reliability across your data stack.
Our promise: we will show you the product.