How TOCA Football Achieved Their Data Quality GOOOOOOAL!
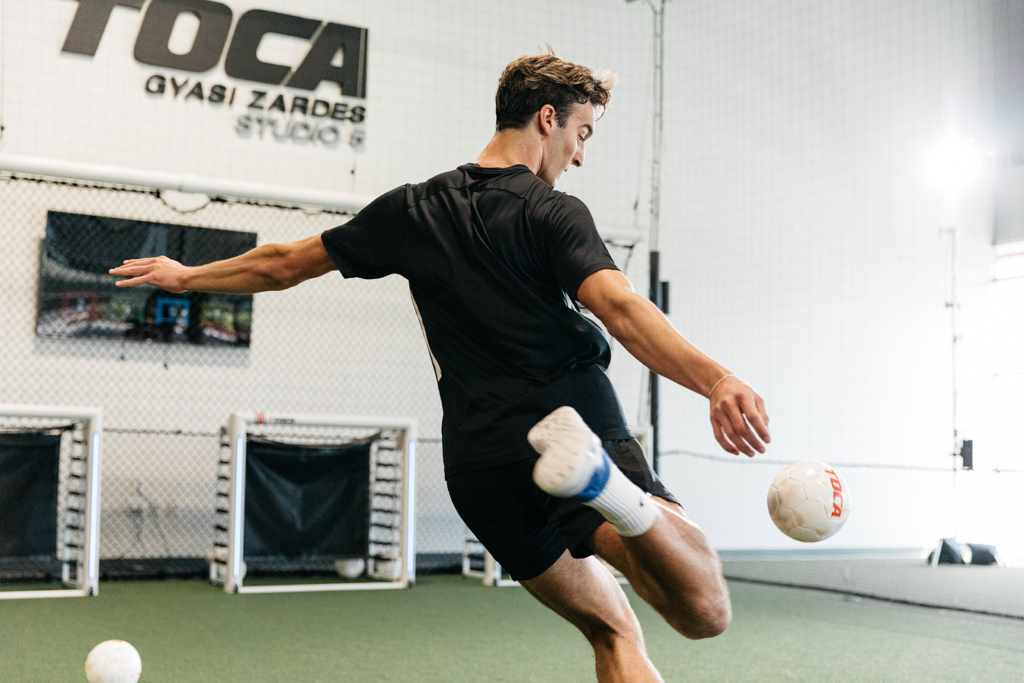
TOCA Football is a technology enabled experience that helps players elevate their soccer game and is an official training partner of Major League Soccer. Their proprietary training system collects data on every session at a ball-by-ball level giving players the insights they need to take their training, and their game, to the next level.
TOCA’s business also needs insights from high-quality data to perform at its peak. Corporate executives and training centers depend on key reports to inform decisions, ensure efficiency, and understand their operations.
TOCA’s data team was able to deliver on those goals and became a trusted teammate by addressing data issues head-on with an assist from Monte Carlo. Here’s how.
Challenge: (Slide)Tackling Data Trust
When Sam Cvetkovski stepped in to lead TOCA’s data team as the director of data and analytics in February of 2022, she inherited a robust modern data stack “roster” consisting of Fivetran, dbt, Snowflake, and Tableau.
Of course, she inherited quite a bit of data debt as well. While the stack was set up well, there was a lot of point-to-point building.
In other words, business questions had been answered with more ad-hoc analysis processes that made it difficult to understand the bigger picture of both the system and the business. On top of that, the data team found themselves playing from the backfoot and having to constantly integrate new data streams from dozens of training centers and disparate point of sale systems.
All of this complexity made it difficult and time consuming to package key metrics into reports with a level of consistency that would imbue data trust.
“For our executive and board reporting we have different KPIs that consist of a certain set of services. We would spend a lot of time with our operational teams going line by line through each service to make sure the numbers were right or if there was a new service we needed to add,” said Sam.
“Data complexity and inconsistency destroy data trust because when you don’t understand the data you don’t trust it. I would get emails from the CEO, president, and others asking where the data is coming from or to explain the logic because there were situations where everything was correct, but numbers wouldn’t match. It got to the point where some people were checking different sources or keeping their own data,” she continued.
While it would have been tempting for the time-strapped team to tackle one challenge at a time, they knew improved coordination with the business, enhanced data quality, and better data model clarity were all related issues that needed to be addressed immediately.
The Solution: Being First to the Ball with Data Observability
Monte Carlo’s data observability platform caught data engineer Shivanshu (Shiv) Gupta’s eye and he brought it to the attention of Sam who had used the solution at a previous company.
“We are too small to be thinking of implementing a custom solution or setting thresholds ourselves. We needed automated data quality monitors–something we could integrate and get value from on day one,” said Shiv, data engineer, TOCA Football.
Other solutions such as Elementary and Metaplane were ruled out for not meeting TOCA’s requirements for data incident impact assessment and end-to-end pipeline coverage.
TOCA started with month-to-month tables based pricing before moving to an annual contract, allowing them to quickly test Monte Carlo’s platform and its value.
“After a few weeks we saw a higher data quality alertness and it was an easy sell,” said Sam. “For example, we have a daily report around our training center performance and we are now able to reach out proactively when those reports are behind or the data is lagging. It is one of those quiet wins when people don’t necessarily realize they don’t have to let us know something is wrong, but we’d much rather be the ones pointing out when something isn’t right with the data.”
Data observability has also led to more efficient and proactive communication with business stakeholders, which has started to restore data trust.
“The alerting in Monte Carlo helped us identify a new service in our POS system, which allowed us to take action to add it to our Lookup tables and prompt our operations team to build a deeper understanding,” said Shiv. “We’d also get alerts when we started getting data from a new center which enabled us to prioritize that integration, rather than being alerted a few days later by stakeholders.”
One of the benefits of data observability has been to standardize processes so they don’t have to code against bad data. It has also made reviewing data with the business much easier. Now instead of a line-by-line review to make sure the data is complete and the numbers are correct, the conversation has advanced to how they should package new services and metrics.
Data observability also played a key role, call it center midfielder, in one of the TOCA data team’s most important initiatives: modeling the business.
“What we had before was an understanding of TOCA pieced together based on how POS system A and POS system B output data. What we’re moving to now–what we call our TOCA data model–starts with how TOCA actually does business,” said Sam.
Shiv was key in transitioning TOCA from a tangled data model to one based on a more traditional Kimball methodology. Monte Carlo was a helpful resource during this process as he reduced clutter and referenced pipeline dependencies.
“I would look from the bottom up. I started in Tableau and removed workbooks that were not getting any eyes. At this point, I’m still being cautious to not delete data or models we might need later,” said Shiv. “At times naming confusion and versioning issues would arise around dbt models, and it was helpful to have Monte Carlo to reference which tables had recent additions and which models were associated with them. It helped us reduce the clutter in our production database.”
As a result of this transformation, the TOCA data team have scaled their efforts so they can place more attention toward athlete performance data. They also proactively created the data consistency, explainability, and accessibility to earn the trust required for making TOCA a leading-edge data-driven organization.
They are fully switching over to the TOCA data model with new dashboards and quality assured business metrics. This has increased their level of data accessibility so they now get questions about how to best use the data rather than comments about not having it.
Results: 20 Data Engineering Hours Saved per Month and a 90% Reduction in Data Downtime
Monte Carlo has helped reduce the data downtime of hundreds of data teams and TOCA Football is no exception. The data team estimates the number of incidents have gone down about 20% as they have implemented the data observability solution and refined their data model.
“We observe these incidents in a better way–a more automated fashion. Over time the alerts have gone down and we’re not hearing about issues from others within the business,” said Shiv.
One of the consequences of TOCAs formerly complex data platform was the elongated time to detect and resolve data incidents. It could be a week or two before an issue was detected and the fix was rarely straightforward.
Today, the TOCA team estimates they can respond to relevant issues within a day and the time to resolution has been reduced by 50%. Whatsmore, the TOCA data team has achieved this increase in data quality while reducing the amount of data engineering hours they spend on data quality by about 13%, or 20 hours a month.
“Our implementation of Monte Carlo was very timely. If we were to go back and evaluate our entire data platform, Monte Carlo was one of the better value adds. Implementation was quick and we saw value fast,” said Sam. “Data observability allowed us to eliminate data distrust and build a partnership with the business so we could be seen as a knowledge center and resource.”
Have a data quality goal of your own? See how your organization can win with data observability by filling out the form below to set up a time to talk with us.
Our promise: we will show you the product.