How Sub-Zero Group Put Data Quality Issues On Ice
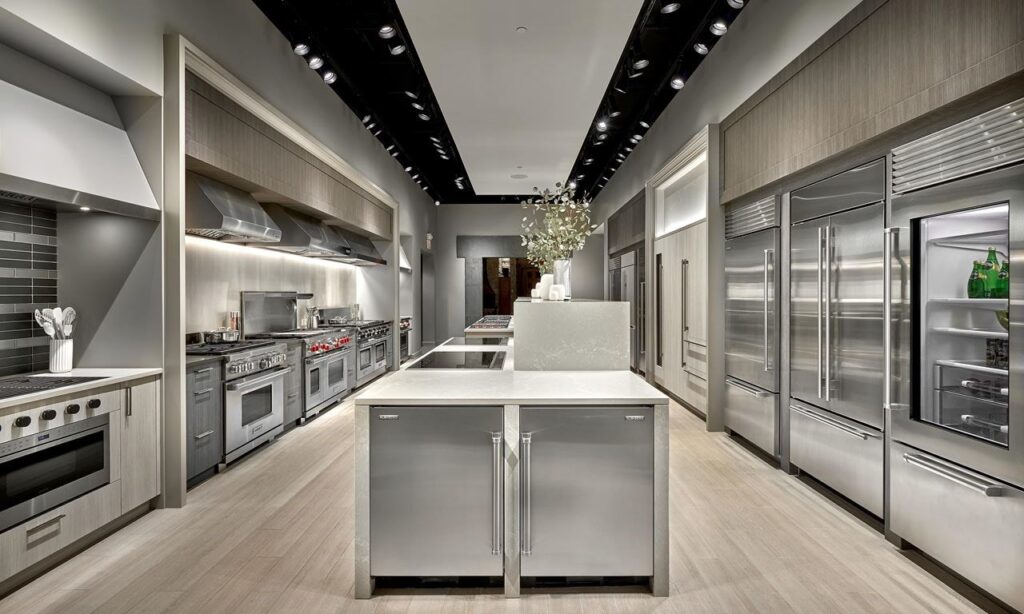
Sub-Zero Group manufactures and sells powerful and dependable kitchen appliances–you won’t find any better. The company has exacting standards for how they manufacture their products, and for how they deliver data products.
In fact, the two are often intertwined. These high-end appliances are also IoT connected devices that, like any modern app, emit data that can be used to provide unparalleled customer service.
Of course, connected devices is just one of several domains–supply chain, sales, and manufacturing are among the others–overseen by the Sub-Zero data team and governed within their Data Governance Program, led by Justin Swenson.
“Each domain has helped us progress in different ways. Sales was among the earliest adopters and helped lead the path to our main analytics stack with Snowflake, Power BI, dbt cloud, and Fivetran,” said Justin. “Whereas manufacturing has helped push for increased standardization and consistency. For that team, it’s important to understand and compare efficiency levels across teams using metrics like takt time, a rate that may be calculated differently depending on the product being built and the process for building it. But for all teams data reliability is paramount.”
Table of Contents
Moving From reactive to proactive
Justin revealed Sub-Zero’s data quality levels have always been relatively high, outside of the periodic pipeline failure that is an inevitable part of life in the modern data stack.
“Our data quality wasn’t bad, but we did have some process issues where a job wouldn’t run and no one would get notified soon enough,” he said. “If that happened on Friday and the data hadn’t refreshed for two or three days that could be a lot for us to backfill. Now, instead of reports going out 6:00 am on Monday, they are processing until noon and folks within the business are starting their week with a feeling of being behind and flying blind.”
Any data quality issue that made its way past the data team chipped away at the faith and trust the data-driven Sub-Zero team had in their reports.
“It doesn’t look good if we receive a complaint from a user on an issue that we were unaware of and it could or should have been caught 6 hours ago,” said Justin.
These types of checks were something that should be automated. And so Justin and the Sub-Zero data team carefully evaluated a half dozen solutions to do just that.
At the start, each solution had a feature that appealed to different personas across the data team. Some saw value in pipeline data differentials, others saw value in hyperscaling code based unit testing. Ultimately, Monte Carlo’s data observability platform was selected over many well-known players in today’s data quality vertical that are focused on the modern data stack for meeting Sub-Zero’s critical requirements for handling data reliability at scale, along with its superior usability.
“Monte Carlo was far enough down the road that they had proven they could do what they promised but still felt very responsive to our feature requests. The solution also was very developer friendly with code-first features, but still had a user-friendly UI so both our technical users and data stewards could leverage it effectively,” said Justin.
Growing trust… and data adoption
Now when data quality incidents occur, not only is the Sub-Zero data team proactively alerted via Microsoft Teams, but they can immediately determine the “blast radius” and warn their data consumers as needed.
Alerts have also created conversations with the stakeholders, helping to enhance collaboration and bridge what can be a communication chasm at many organizations.
“We’re gradually digging into real issues. An anomaly might trigger on a specific metric, and while today it looks more like KPI threshold monitoring than actual data quality monitoring, it’s a starting point,” he said. “It creates a conversation that can quickly transform into discussions around a new sensor type, the data we expect to get from it, and what failures they experience.”
This broad visibility and deep coverage has increased data trust not just with consumers, but other members of the data team as well leading to more streamlined processes.
“Our centralized data team lives in dbt and leverages those alerts, but they no longer need to build out custom dbt tests in every model. They’re learning that Monte Carlo can handle to task and keep their quality checks in one tool,” said Justin. “It’s also been helpful for maintaining quality levels and institutional knowledge as we’ve onboarded new data engineering team members.”
Sub-Zero has also achieved a 90% incident status update rate, choosing to temporarily centralize the incident management process with Justin as the main incident dispatcher. His in-depth knowledge of the data sets and business operations has assisted in these efforts.
“I saw one of our supply chain tables that normally gets around 1,000 rows per run receive 45,000 and shot that off to the team to investigate further, but I’m able to quickly mark other volume anomalies as expected because I know for those tables the data volume is dependent on the cadence of our product line shift schedules and line run times,” Justin said.
As a result, Sub-Zero has a clear view of its data health at both the organizational and domain levels.
“This way there is no noise. The engineers and stewards see and pay attention to things that matter to them,” he said.
In these ways Monte Carlo has been able to make the lives easier not just for Justin’s data governance team, but for data consumers, analytical engineers, data stewards, and of course troubleshooting data engineers.
Eye on the future of data quality
One of the Sub-Zero data team’s main priorities is to consolidate source systems and move more of the generated data into Snowflake.
This has historically been a challenge as the data within these source systems has been messy and ungoverned. But with Monte Carlo, this project now has a safety net.
“We had a multitude of different customer-focused CRM-type applications and now we are moving towards one, but the data is still all over the place. Once it is moved into the warehouse where Monte Carlo can track it, we can get a better understanding of the quality, which will give us peace of mind,” said Justin. “Then we can certify it as good quality data and that starts to allow us to go further upstream to clean up some of our processes to improve the data quality and technical debt on the source system side.”
And while more data is being moved into the main analytical stack and observed, Monte Carlo has also extended its monitoring capabilities to observe more of Sub-Zero’s data stack.
“As Monte Carlo moves into SQL Server and Oracle it will help us find problems at the root layer even more effectively, which is really taking us in the right direction,” he said. “The future looks good. We have progressed so much, but in some ways I feel like we are just getting started.”
Our promise: we will show you the product.