How Contentsquare Reduced Time to Data Incident Detection by 17 Percent with Monte Carlo
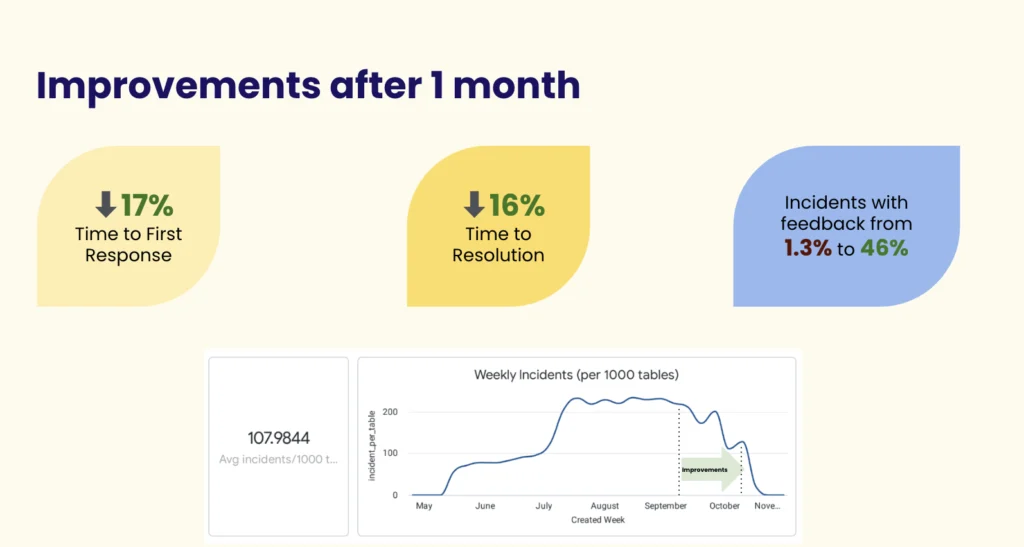
Like many hyper growth startups, Contentsquare is all about data.
The company leverages AI to provide its users with actionable, data-driven insights about their customers, which empowers teams to improve the experience for users of their websites and digital apps.
Founded in Paris a decade ago, Contentsquare today is a fast-growing global company that just completed its Series F funding round in July of 2022. The company of 1,600+ employees serves over 1,000 clients, and its teams churn through massive volumes of data on a daily basis.
Contentsquare’s Data Governance team, which includes Global Data Governance Lead Otávio Bastos and Data Governance Strategist Kenza Zanzouri, governs the access and application of data at Contentsquare, and ensuring data reliability falls under their remit.
“Our purpose as a team is to make data accessible, understandable, and reliable,” says Otávio.
As a hyper-growth startup, Contentsquare leverages data across dozens of critical use cases, and the team requires reliable performance monitoring in order for its core business functions to operate.
“When we say fast-growing, we have skyrocket growth,” explains Otávio. “The result [of that growth] is that we need near real-time business performance monitoring. And the challenge there is that teams are evolving so fast, new processes are being created, new data is evolving.”
Otávio and his team treat every team output as a data product. Each data product is linked to use cases, which are linked to underlying data. Data quality monitoring underpins all that underlying data, and the data team conducts regular checks to ensure each data product works as designed.
As the company scaled, Contentsquare needed to implement a quick-value solution that could help them build the foundation of a reliable data platform.
Contentsquare’s existing stack includes multiple elements in various layers. On the information system side, Salesforce, Workday, and Hubspot serve as data sources. An integration layer by Matillion orchestrates the data, which is then stored in a Snowflake-powered data lake, data warehouse, and data mart.
Every piece of Contentsquare’s data is linked to a searchable catalog created by Atlan. “Any time any employee needs data KPIs or dashboards, they go to a central tool and search,” says Otávio.
Finally, all this data flows to a data visualization layer facilitated by Tableau and Data Studio.
Because so many teams at Contentsquare rely so heavily on data and can access it via the Atlan catalog, it is imperative that team members trust the integrity of that data. Before implementing Monte Carlo, the Data Governance team did not have that trust.
Here’s how they gained it.
The challenge: lack of stakeholder trust in data
Otavio and his team needed three overarching things:
1) To ensure high-quality, usable data the business teams trusted
2) To shift away from time-consuming manual data quality checks
3) To institute a seamless data incident resolution process
Each of these components was particularly necessary in this phase of hyper growth at Contentsquare.
“We have to balance creating value versus building foundations for reporting scalability,” says Otávio.
Prior to implementing Monte Carlo’s data observability platform, the team was inundated by writing data checks.
“We had too many manual data checks by operations and data analysts,” says Otávio. “It was very time-consuming. We had low efficiency on internal reporting, and it took a lot of time to build new reports on new metrics.” This inefficiency applied pressure to the data team, where FTEs were spending hours each week on quality check, without gaining full coverage as the business grew.
The company also faced dashboard downtime, as data issues caused certain data dashboards to be unavailable. These data downtime issues often took days or even weeks to identify and resolve. As a result, stakeholders didn’t trust data and wouldn’t use it—which further exacerbated pressure on the data team.
The solution: End-to-end data observability with Monte Carlo
Contentsquare partnered with Monte Carlo to overcome its data governance challenges. By deploying Monte Carlo’s end-to-end data observability tool, Contentsquare expedited data quality monitoring, cemented business-wide trust in the data, and crafted a seamless, collaborative incident resolution workflow with the data team.
After implementing Monte Carlo, the data team shifted from burdensome manual checks to automated data quality coverage that required zero manual configuration.
“We just say to Monte Carlo, ‘I want to check for anomalies on this table,’” says Otávio. “We have some kind of unsupervised monitoring, and we can automatically start detecting some very important issues that cannot be detected by human beings.”
Monte Carlo also enables automatic business rule checks.
“It’s pretty much like SQL monitors, where we define a perfect behavior for the data on the business side,” says Otávio.
With Monte Carlo, the team can easily check how data aligns with these rules. Further, the team can leverage Monte Carlo to monitor metrics. “We can calculate any KPI using SQL codes, and we can use Monte Carlo to verify if [a certain] metric is increasing or decreasing faster than normal standards,” says Otavio.
Comprehensive visibility into their data ecosystem
Monte Carlo’s ability to integrate easily with Contentsquare’s existing systems means that data domain owners have comprehensive visibility over the data they use.
“Everything is linked,” says Otávio. “Any anomaly that we detect is automatically sent by Slack to the appropriate people that we have configured.”
These alerts are also automatically sent to Contentsquare’s searchable data catalog, so all employees are immediately aware of any data issues Monte Carlo might reveal. That workflow engenders trust in the KPIs generated by SQL queries, which empowers data users to feel confident that their data is reliable.
Finally, deploying Monte Carlo enabled the data team to create an incident resolution process that allowed them to identify and resolve issues more quickly. With lineage embedded directly within the incident resolution workflow, the data team could more easily understand which data in the pipeline mattered most and resolve any issues accordingly.
The outcomes: 17% reduction in data incident detection time; 16% percent reduction in time to resolution
Partnering with Monte Carlo strengthened the culture around data use at Contentsquare. Implementing a comprehensive data observability platform expedited data incident detection and resolution, reduced alert fatigue, and cultivated a culture of knowledge-sharing about data health and incident resolution.
Deploying Monte Carlo led to a 17% reduction in data incident detection time and a 16% reduction in time to resolution – in just one month.
“We really reduced drastically this time to the first response,” says Otávio.
Monte Carlo didn’t just help the data team detect and solve incidents more quickly—it also helped forge a new kind of culture around data quality and observability.
Data incident documentation increased by 46% with Monte Carlo—and that institutional knowledge-sharing helps the team gain a more comprehensive, nuanced understanding of the company’s data health.
“We can see it’s working, we can see people are solving [issues] faster, we can see they’re starting to engage,” says Otávio. “We can see they’re starting to align themselves within every department to tackle these issues and create a better data environment for analysts to exploit data, create dashboards, create analysis, correlations, models, or any data product.”
Clear data quality ownership
Prior to implementing Monte Carlo, teams suffered from “the pressure that is caused by rule overruled, not specifically targeted at the right audience,” according to Kenza. A lack of clear data quality issue ownership compounded this problem.
“Both challenges are very correlated,” explains Kenza. “Identifying the right person for each specific data quality rule can reduce stress, ease the adoption, [and] address all Monte Carlo incidents correctly in a timely manner.”
Monte Carlo streamlined the incident detection workflow, so a single owner received a single alert. “Clear ownership in data governance can be a game-changer for data quality,” says Otavio. In order to ensure incident resolution, Contentsquare realized it needed to define exactly who was responsible for each issue—and Monte Carlo helped facilitate that ownership.
Ultimately, Monte Carlo helped Contentsquare build a solid data reliability foundation that would allow the company to scale more comfortably and confidently.
“Sometimes when we are going so fast, we tend to focus only on the value creation: new dashboards, new models, new correlations with new data explorations,” says Otávio. “We forget to put in place good data engineering, good data governance, and an efficient data analytics team. This is very important to make sure in the long term we are scalable, and we can do more with the same team in the future.”
Creating systems and processes that made data incidents visible to multiple teams and assigned clear ownership over their resolution strengthened data governance at Contentsquare. “Data governance is to give more trust to all of our data, to give more visibility, and also to be able to have better communication between departments,” says Kenza.
Otávio takes it a step further.
“By anticipating and communicating to the people who are interested in [data quality information], we actually created a revolution,” he says. “Now, we can sleep tight at night. We know if anything happens with our data, we’re going to be alerted before our end users of our dashboard[s] see any problem.”
Ready to start your own data reliability revolution? If you’re interested in scaling data trust across your organization with end-to-end data observability, don’t wait—reach out to Monte Carlo to learn more.
Our promise: we will show you the product.