Data Observability: How Yotpo Fixes Data Quality at Scale with Monte Carlo
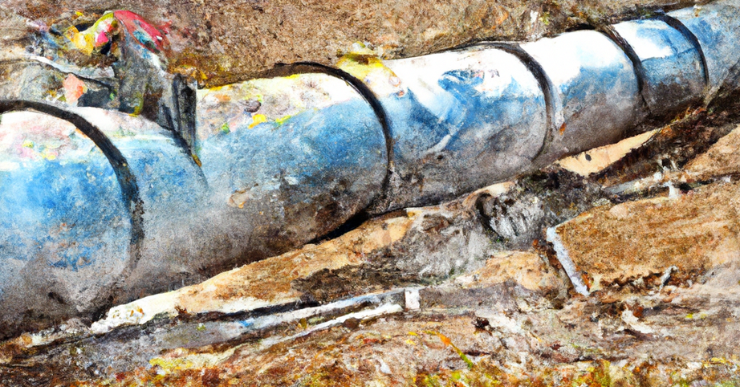
Companies spend upwards of $15 million annually tackling data downtime, in other words, periods of time where data is missing, broken, or otherwise erroneous, and 1 in 5 companies have lost a customer due to data quality issues.
Fortunately, there’s hope in the next frontier of data: observability. Here’s how data engineers and BI analysts at Yotpo, a global eCommerce company, increases cost savings, collaboration, and productivity with data observability at scale.
Yotpo works with eCommerce companies across the world to help them accelerate online revenue growth through reviews, visual marketing, loyalty and referral programs, and SMS marketing.
For Yoav Kamin, Director of Business Performance, and Doron Porat, Data Engineering Team Leader, having consistently accurate and reliable data is foundational to the success of this mission.
The challenge: broken data pipelines & dashboards
Since day one, Yotpo has invested in a distributed data platform for internal teams that support the company’s diverse data needs, from generating marketing reports to empowering product development teams to build better services for their users.
Over the past few years, Yotpo has grown exponentially, expanding their operations globally and acquiring companies including Swell Rewards and SMSBump. As Yotpo grew, so too did the number of data sources and complexity of their data pipelines. Over time, it became harder to keep track of data completeness, lineage, and quality, three critical features of reliable data. This data downtime, in other words, periods when data is missing, inaccurate, or otherwise erroneous, led to time-intensive and costly data fire drills that caused friction between Yotpo’s Business Performance and Data Engineering teams.
“Time and again, our staff would approach my team and tell us the data is wrong, but we had no idea how the data broke in the first place,” said Doron. “It was clear to us that we had to gain better control over our data pipelines, as we can’t have our data consumers alerting us on data issues and keep getting caught by surprise , no one would be able trust our analytics this way.”
To tackle this problem, Yotpo needed a better way to manage data health and discovery. At the end of the day, they required a solution that would empower them with the right information at the right time to alert for and prevent abnormalities in their data pipelines and business intelligence dashboards, before they impacted the business.
The solution: data observability with Monte Carlo
To help them eliminate data downtime and unlock the potential of their data, Yotpo chose to work with Monte Carlo because of their proactive approach to preventing broken data pipelines through end-to-end data observability.
The Monte Carlo Data Observability Platform automatically monitors across key features of Yotpo’s data ecosystem, including data freshness, distribution, volume, schema, and lineage. Without the need for manual threshold setting, Monte Carlo quickly answers such questions as:
- When was my table last updated?
- Is my data within an accepted range?
- Is my data complete? Did 2,000 rows suddenly turn into 50?
- Who has access to our marketing tables and made changes to them?
- Where did my data break? Which tables or reports were affected?
Monte Carlo’s no-code integration and 20-minute onboarding meant Yotpo was up and running with data observability in minutes, not weeks or months.
Outcome: Cost savings by catching data anomalies in their tracks
Out-of-the-box, the platform gave Yotpo an overview of their Redshift environment, including all data assets, schemas, and tables. Their machine learning algorithms automatically generated rules to inform data downtime monitoring and alerting, providing immediate value.
A recent example of Monte Carlo and data observability’s impact for the team was when an erroneous data point in their Segment instance generated 6x more rows than expected, even with seasonality and normal data fluctuations taken into account. Image courtesy of Monte Carlo.
“When the spike happened, Monte Carlo alerted my team immediately, allowing us to investigate and troubleshoot the anomaly before it affected downstream data consumers on the Marketing team,” said Doron. “Since we caught this right away, I was able to rest assured that no important business metrics would be impacted. There is no way we would have known if anything went wrong if it wasn’t for Monte Carlo.”
Since the Data Engineering team was notified of the issue before it affected their stakeholders, they were able to fix their pipeline and prevent future anomalies from jeopardizing the integrity of their data.
Outcome: Improved collaboration by tracing field-level lineage
Another use case where data observability became crucial to Yotpo’s day-to-day operations was when Yotpo’s Business Applications team, the group responsible for integrating and maintaining internal operational systems such as Salesforce, wanted to replace an outdated field with a new one. Many of their dashboards heavily relied on this field so they had to prepare in advance for this change.
Using information-rich query logs, Monte Carlo helped Yotpo understand 1) what are the downstream dependencies for this field, 2) who is using the table and how 3) which dashboards are currently using the old field (lineage) and 4) where the new field had been added to track the progress of this update. Image courtesy of Monte Carlo.
“Monte Carlo’s lineage highlights upstream and downstream dependencies in our data ecosystem, including Salesforce, to give us a better understanding of our data health,” said Yoav. “Instead of being reactive and fixing the dashboard after it breaks, Monte Carlo provides the visibility that we need to be proactive.”
Outcome: Increasing productivity by keeping tabs on deprecated data sets
Additionally, Monte Carlo gives Yotpo greater transparency into the relevancy and usage patterns of important data assets, informing them when different attributes (such as record type ID and specific data sets) are deprecated. The Monte Carlo data catalog functionality lets them track where new fields are being added and used so you can keep tabs on what dashboards need to be updated. This knowledge ensures Yotpo can trust their data to be accurate and reliable, even as their data platform evolves.
“Once you lose trust in your data, you lose reliability,” said Doron. “With Monte Carlo, there’s less data downtime and more data reliability. Long gone are the days of painful schema changes and broken dashboards.”
Impact of Data Observability at Yotpo
For Yotpo, Monte Carlo’s approach to data observability empowered them to solve data quality issues fast so they could start trusting their data to deliver reliable, actionable insights for the business.
“Our execs rely on my team’s dashboards to make decisions. With Monte Carlo, we know exactly what to update when there’s a change in our data, so there’s no downtime and no fire drills. Our decision makers are happier and I can sleep at night,” said Yoav.
Among other benefits of data observability, Monte Carlo has enabled Yotpo to:
- Increase cost savings by reducing time to resolution of tedious data fire drills and restore trust in data for vital decision making
- Better collaborate between data engineering and data analyst teams to understand key dependencies between data assets
- Drive greater efficiency and productivity by gaining end-to-end visibility into the health, usage patterns, and relevancy of data assets
With Data Observability in tow, Yoav, Doron, and the rest of the team at Yotpo are well-prepared to eliminate data downtime and continue unlocking the potential of their data.
Special thanks to Yoav, Doron, and the rest of the Yotpo team!