How to Calculate the Cost of Data Downtime
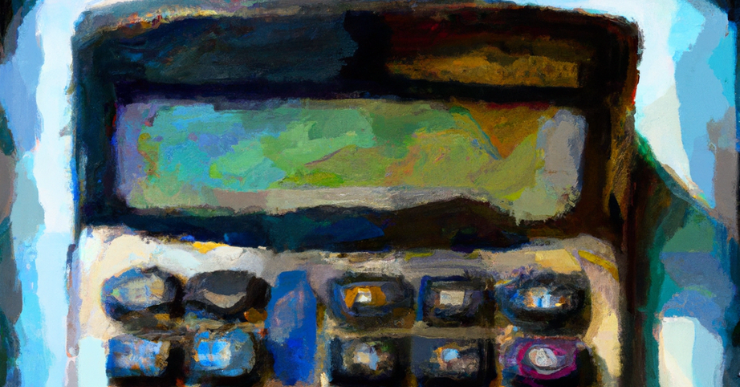
In addition to wasted time and sleepless nights, data quality issues lead to compliance risks, lost revenue to the tune of several million dollars per year, and erosion of trust — but what does bad data really cost your company? I’ve created a novel data downtime calculator that will help you measure the true cost of data downtime on your organization.
What’s big, scary, and keeps even the best data teams up at night?
If you guessed the ‘monster under your bed,’ nice try, but you’d be wrong. The answer is far more real, all-too-common, and you’re probably already experiencing it whether or not you realize it.
The answer? Data downtime. Data downtime refers to periods of time when your data is partial, erroneous, missing, or otherwise inaccurate, ranging from a few null values to completely outdated tables. These data fire drills are time-consuming and costly, corrupting otherwise excellent data pipelines with garbage data.
In this post, we’ll look at the cost of data downtime, how to calculate it, and what you need to know to get started.
Table of Contents
The true cost of data downtime
One CDO I spoke with recently told me that his 500-person team spends 1,200 cumulative hours per week tackling data quality issues, time otherwise spent on activities that drive innovation and generate revenue.
To demonstrate the scope of this problem, here are some fast facts about just how much time data teams waste on data downtime:
- 50–80 percent of a data practitioner’s time is spent collecting, preparing, and fixing “unruly” data. (The New York Times)
- 40 percent of a data analyst’s time is spent on vetting and validating analytics for data quality issues. (Forrester)
- 27 percent of a salesperson time is spent dealing with inaccurate data. (ZoomInfo)
- 50 percent of a data practitioner’s time is spent on identifying, troubleshooting, and fixing data quality, integrity, and reliability issues. (Harvard Business Review)
Based on these numbers, as well as interviews and surveys conducted with over 150 different data teams across industries, I estimate that data teams spend 30–40 percent of their time handling data quality issues instead of working on revenue-generating activities.
The cost of bad data is more than wasted time and sleepless nights; there are serious compliance, financial, and operational implications that can catch data leaders off guard, impacting both your team’s ROI and your company’s bottom line. (New: see just how much with our data quality value calculator.)
How data downtime impacts businesses
Data downtime isn’t just an inconvenience—it also has very serious real-world implications for both data teams and the businesses they support.
From lost revenue to increased exposure to risk, let’s take a look at some of the most common costs associated with data downtime .
Compliance risk
For several decades, the medical and financial services sectors, with their responsibility to protect personally identifiable information (PII) and stewardship of sensitive customer data sources, was the poster child for compliance.
Now, with nearly every industry handling user data, companies from e-commerce sites to dog food distributors must follow strict data governance mandates, from GDPR to CCPA, and other privacy protection regulations.
And bad data can manifest in any number of ways, from a mistyped email address to misreported financials and can cause serious ramifications down the road; for instance, in Vermont, outdated information about whether or not a customer wants to renew their annual subscription of a service can spell the difference between a seamless user experience and a class action lawsuit. Such errors can lead to fines and steep penalties.
Lost revenue
It’s often said that “time is money,” but for any company seeking the competitive edge, “data is money” is more accurate.
One of the most explicit links I’ve found between data downtime and lost revenue is in financial services. In fact, one data scientist at a financial services company that buys and sells consumer loans told me that a field name change can result in a $10M loss in transaction volume, or a week’s worth of deals.
Behind these numbers is the reality that firefighting data downtime incidents not only wastes valuable time but tears teams away from revenue-generating projects. Instead of making progress on building new products and services that can add material value for your customers, data engineering teams spend time debugging and fixing data issues. A lack of visibility into what’s causing these problems only makes matters worse.
Erosion of data trust
The insights you derive from your data are only as accurate as the data itself. In fact, it’s my firm belief that numbers can lie and using bad data is worse than having no data at all.
Data won’t hold itself accountable, but decision makers will, and over time, bad data can erode organizational trust in your data team as a revenue driver for the organization. After all, if you can’t rely on the data powering your analytics, why should your CEO? And for that matter, why should your customers?
To help you mitigate your data downtime problem, we put together a Data Downtime Cost Calculator that factors in how much money you’re likely to lose dealing with data downtime fire drills at the expense of revenue-generating activities.
The best way to calculate data downtime
The formula to calculate the cost of data downtime is actually relatively simple. The annual cost of your data downtime can be measured by the engineering time lost and other resources spent to resolve it.
I’d propose that the right data downtime calculator factors in the cost of labor to tackle these issues, your compliance risk (in this case, we used the average GDPR fines), and the opportunity cost of losing stakeholder trust in your data. Per earlier estimates, you can assume that around 30 percent of an engineer’s time will be spent tackling data issues.
Bringing this all together, your Data Downtime Cost Calculator is:
Labor Cost: ([Number of Engineers] X [Annual Salary of Engineer]) X 30%
+
Compliance Risk: [4% of Your Revenue in 2019]
+
Opportunity Cost: [Revenue you could have generated if you moved faster, releasing X new products, and acquired Y new customers]
= $ Annual Cost of Data Downtime
Keep in mind that this equation will vary by company, but we’ve found that our framework can get most teams started.
Measuring the cost of your data downtime is the first step towards fully understanding the implications of bad data at your company. Fortunately, data downtime is avoidable. With the right approach to data reliability, you can keep the cost of bad data at bay and prevent bad data from corrupting good pipelines in the first place.
The answer to data downtime is data observability
As you can see from the calculations above, data downtime is no small problem. And as your data needs grow, your data downtime fire drills will too.
The key to reducing data downtime is implementing an end-to-end data quality solution that can scale with your data environment. Data observability provides the programmatic automation and comprehensive quality coverage data teams need to be able to detect and resolve incidents fast.
Neutralize the impact of data downtime with data observability.
Have another way to measure the impact of data downtime? We would love to hear from you. Book a time to speak with us using the form below.
Our promise: we will show you the product.