5 Ways Generative AI Changes How Firms Approach Data (And How It Doesn’t)
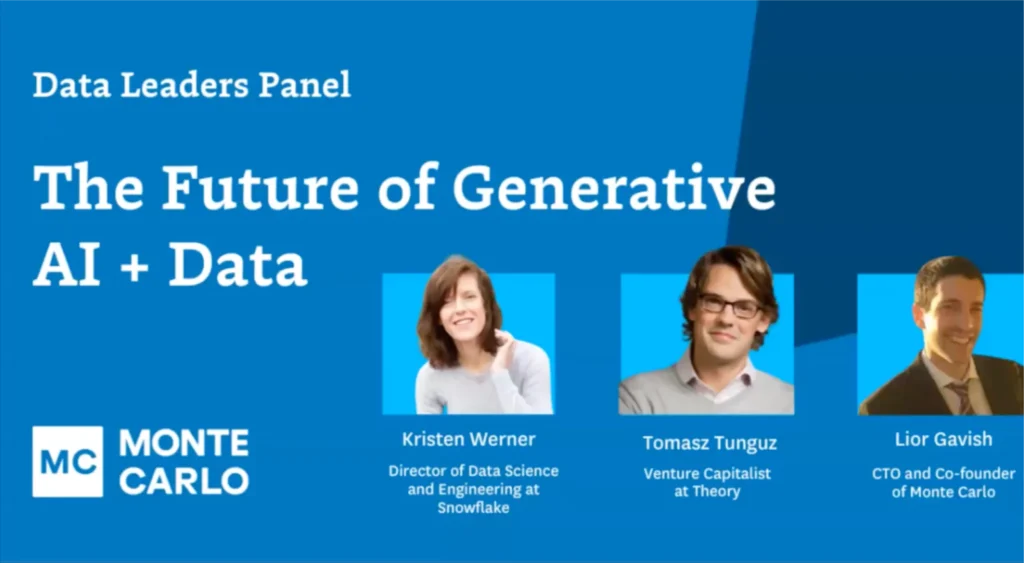
Generative AI is not a new concept. It’s been studied for decades and applied in limited capacities. That is until ChatGPT shocked and awed our collective consciousness in late 2022.
Still, generating a recipe for lasagna is an entirely different process than infusing generative AI capabilities across a business or integrating large language models (LLMs) into data engineering workflows.
Change is coming, but what will the impacts be for how organizations approach data and what hurdles still need to be overcome? To answer that, we convened:
- Kristen Werner, Director of Data Science and Engineering, Snowflake
- Tomasz Tunguz, General Partner, Theory Ventures
- Lior Gavish, co-founder CTO, Monte Carlo
Collectively their experience includes creating new technologies, funding a bevvy of GenAI startups, and helping thousands of customers leverage AI to get more value from their data.
While their estimates varied from 50 to 20% on the “how much of generative AI is hype” spectrum, every panelist agreed this transformational technology had practical applications today and held tremendous potential. So let’s take a look at some of the recurring themes.
In This Article:
5 generative AI benefits

When it comes to Gen AI, it’s difficult to separate fact from fiction and practical application from snake oil. Here are some current and likely ways generative AI is contributing value to organizations and data teams both today and in the near future.
#1- Increasing data accessibility
The lowest hanging fruit for generative AI within the world of data? The ability for non-technical users to enter natural language prompts that can generate SQL queries to retrieve specific data points.
“Text to SQL is ubiquitous,” said Tomasz. “OpenAI is developing it. You have it in the BI layer, you have it in data exploration tools. I think that’s very low hanging [fruit].”
Automating SQL queries can radically democratize data accessibility for non-technical users, and thus accelerate time-to-value.
“I think AI opens up the possibility of people that are not data proficient …that can actually get access to meaningful data, meaningful insights,” said Lior. “And even for people that do know SQL, it’s going to accelerate that process. So we’re just going to be getting data in the hands of more people.”
Data platforms like Snowflake are investing in how generative AI can not just generate SQL, but re-imagine the user-data experience. Kristen talked about how one of the data cloud’s more experimental features, currently in development, aims to link LLMs, SQL queries, and data visualization.
“The value chain can take quite a long time to generate the right data and then get a satisfactory end user experience. Is it a push notification? Is it a dashboard? Is it a BI report?” she said. “I’m really excited about the ability for AI to streamline some of these steps and allow end users to reach more vertically into the stack more quickly with a [better] end experience… It’s not today, but I think it will come to be.”
#2- Extracting insights from data and chart analysis
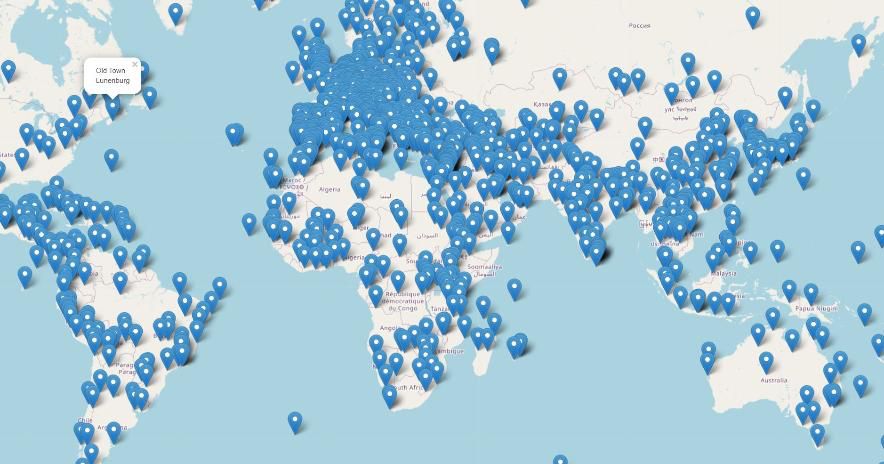
Generative AI can make an impact beyond just querying and visualizing data by taking the next step and extracting insights from it. In fact, that’s a process already employed by Tomasz.
“ChartGPT is a specialized product focused just on charting data. And if anybody’s played with [ChatGPT] Code Interpreter, it’s pretty crazy,” he said. “You can take an Excel spreadsheet of a publicly traded company, upload it into Code Interpreter and ask it to summarize the state of ‘Google after earnings’ and it will produce some pretty significant insights.”
#3- Putting unstructured data to work
All of our expert panelists were excited about the potential for generative AI to enable data teams and organizations to extract value from non-relational sources.
“There’s plenty of unstructured data in the world. Text and images that require specialized skills and tools to analyze it that [are resource constrained],” said Lior. “You can imagine Gen AI extracting structure from those textual and image data sets and putting it to work in the same way that we’ve been able to put relational data to work.”
Tomasz referred to this process as “information fracking.”
“At Theory [his venture firm], we have something like 10,000 documents that we’ve collected on startups…. And about two weeks ago, we produced our first investment memo summary on a space that’s called generative RPA. And it was all produced just by fracking this information with lots of text files,” he said.
Snowflake and other data platforms are releasing features that will accelerate the ability of data team’s to make this a reality.
“Document AI is a growing project as a result of our acquisition of Applica and that use case is really around document reading and interpreting documents,” she said. “This could be for HR for procurement contracts, and that sort of thing…. The customers I meet are sitting on a bunch of [data] and they want to get value out of it.”
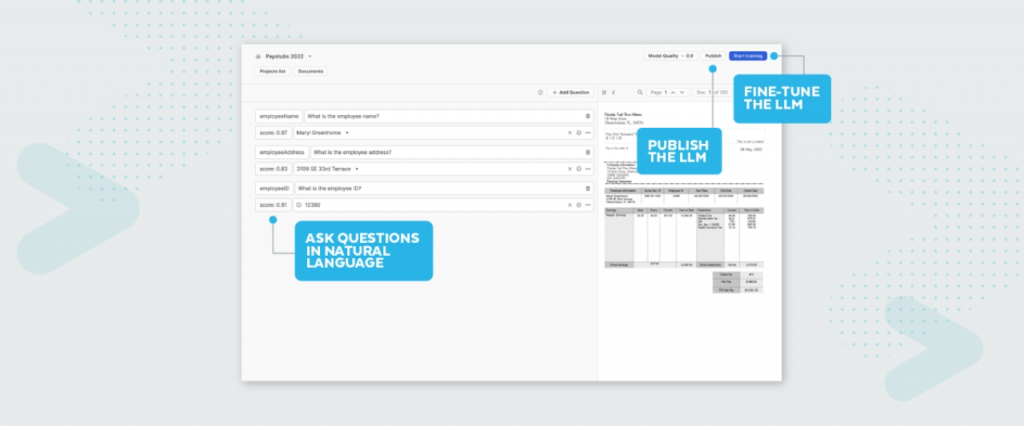
#4- Accelerating data pipeline development
Generative AI may or may not revolutionize data pipeline architecture, but it will certainly allow data engineers to build and deploy them more quickly.
“Gen AI is already very good at writing SQL, Python, Scala or whatever it is that you’re writing your pipelines in. And so we’re going to see GenAI making engineers more effective and faster in building those solutions,” said Lior. “I’m not sure it’s 10X more effective..but it’s certainly 20% or 30% more effective and that’s meaningful for a lot of teams.”
Lior was quick to point out, however, that it’s unlikely data modeling and understanding how data sources fit together would be an activity performed by AI saying, “I think that’s still going to be done by humans for the foreseeable future.”
#5- Cutting costs (and maybe revenue generation)
It’s a cost cutting environment, and many organizations are prioritizing how generative AI can help with those initiatives.
“There are two ways to go about improving profitability. One is on the revenue side, which is much more external facing and where you’re going to run into a lot of issues,” said Kristen. “The other [way to improve profitability] is to reduce cost internally…A lot of people are asking, ‘how can we reduce internal costs using AI?’ Then hopefully [there are] a lot of lessons learned that can help build the path to more external facing and revenue generating use cases.”
5 generative AI challenges
Now that we’re all drunk on the possibilities of AI, it’s time to sober up with some very real challenges and constraints. These include:
#1- Hallucinations, reliability, and trust
It’s now fairly well understood that large language models hallucinate, or in other words, confidently produce incorrect results. This can be challenging as we often conflate well reasoned arguments with correct ones.
“We’ve seen some of the challenges, whether it’s hallucinations or the challenges around making GenAI work in very specific domains that are not general purpose…I think all these things are still significant challenges,” said Tomasz.
It’s not just hallucinations however. Like analytical dashboards and machine learning applications, generative AI models will only be as reliable and trustworthy as the underlying data it is accessing or being trained on. These aren’t new problems, but they will be more prevalent and require more scalable solutions.
“Choosing what data set to use, how to validate it, how to clean it, and how to put it together, to answer the business questions [when you have] fewer analysts in the loop is going to exacerbate some of the governance issues that exist today,” said Lior. “How do you identify what data sets are trustworthy, which ones are useful, and how do you have the right metadata and documentation to make the data useful for a model?”
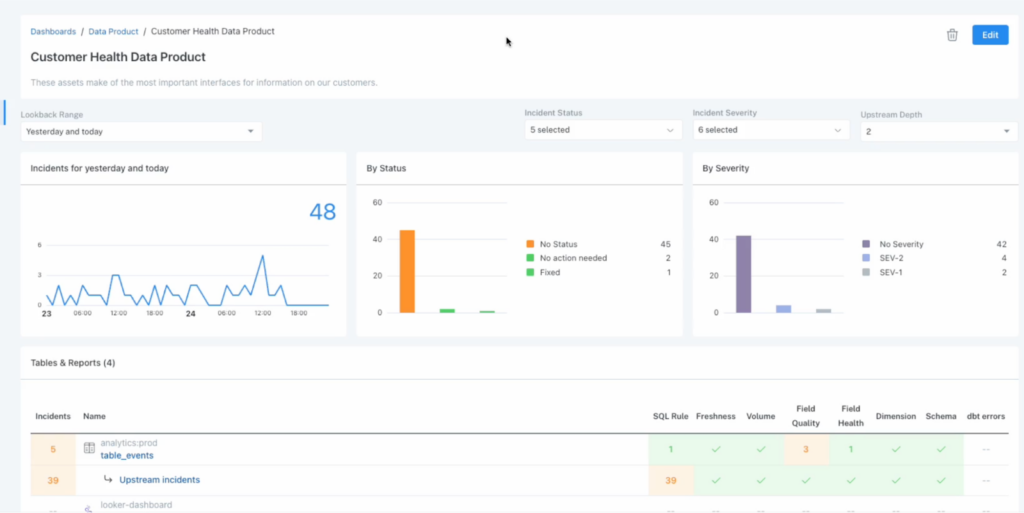
The underlying data stack will also serve as a key guide for generative AI to understand the underlying data.
“If you’re asking the model how to interpret your business based on what it knows about the rest of the world, well, the rest of the world doesn’t know how you interpret your business,” said Kristen. “Where do you impart a business logic into your data stack that serves every subsequent layer? I think having curated some key rules around your business, whether it’s entity related or relationship embedded, at the ground layer of your data stack is gonna serve both [model speed and accuracy].”
#2- Solving its own problems
Tomasz shared a relevant experience on the challenges of using generative AI for more expansive tasks:
“I was preparing a presentation and I wanted to chart the GitHub star growth of a particular GitHub repository. And so there’s two or three pieces of code that I needed to build. So I asked the robot to produce a crawler to get the information. And then another part was to actually plot it, and then I wanted to automate it so that I could give it a list of repositories to go through,” he said. “It had a really hard time taking a big chunk of a problem like that and decomposing it into individual tasks and then building it up.”
He also expressed frustration with Gen AI models’ tendency to find themselves backed into corners once they have made a mistake.
“[When] it goes down a wrong path where the code doesn’t execute, it has a really hard time forgetting the mistake that it made and it wants to continue to improve and you have to restart the session. It has a very, very persistent memory,” he said.
#3- Security and privacy
This is probably the biggest obstacle to widespread adoption of Gen AI according to Tomasz.
“Many [banks and companies in the Fortune 2000] have just blocked [Gen AI] all together. I think a lot of them are waiting for AI products to be shipped within an Azure VPC so they can control it,” he said. “In the cloud, we moved to multi-tenancy where multiple customers are on the same machine…With LLMs, will customers accept that or do we actually need a LLM on a per client basis?”
The industry is in the early stages of navigating these questions, but so far there have been four primary ways of solving these security and privacy challenges:
- Banning it
- Periodically telling the LLM to “forget all my data and don’t use it to train”
- Using an intermediate layer to intercept personally identifiable information
- Accepting the risk
The solution is often dictated by the use case. Generating a templated website has much less risk than customer service where customer information and credit cards may be processed.
And of course, it wouldn’t have been a generative AI discussion if the topic of prompt injection and a relevant xkcd on SQL injection hadn’t come up:
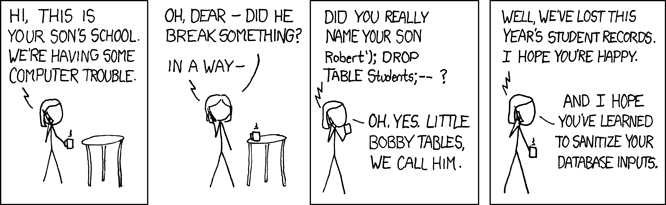
#4- We’re early on infrastructure and solutions
Navigating governance, reliability, security, and other generative AI challenges will require a robust infrastructure that doesn’t quite exist today.
“It’s still the early days on what I’ll call the infrastructure and solution side. When we talk about Gen AI right now, for most people, that means using OpenAI’s APIs. A select few might be playing around with some open source models and vector databases, but I think the tooling around it is fairly early on,” said Lior.
Classification and access control are two other challenges where supporting infrastructure can help.
“Data classification is a hard problem. When you think about access controls and then access controls at scale…you have to already have an understanding of classification,” said Kristen. “We’ve been spending a lot of time in this space and have some features that are native to Snowflake as well.
PII is actually not so hard to figure out, but then I think there’s a focus on enabling people to create custom classifiers. Because the universe is unlimited and if you [think] about what constitutes trade secrets or customer data, that can be dynamic within a company.”
#5- Skill set shifts
Perhaps the longest portion of the discussion centered around both the demand for emerging generative AI skill sets as well as the workforce shifts that will result from deploying these large language models across the enterprise.
The big winners? Data teams who will see an exponential demand for their services, particularly for evaluating and fine tuning different generative AI models.
“I think some [responsibility] will shift to the data team because they’ll need to ensure the outputs are accurate and the solutions that are being used are in compliance with whatever the regulation is applied to that business,” said Tomasz. “And they’ll be the ones who will be responsible for the initial evaluation all the way through the ongoing maintenance. So I think that data teams will growteams grow.”
Lior pointed out that past technology advances indicate that it’s unlikely there will be fewer data people, but rather we will generate more value from data.
“We’ve seen it time and time again with software in the past. We eventually got from Assembly to Python, which is way more productive. But did it mean that we had fewer software engineers? No, we had more of them and we had more software in the world and I think that’s for the foreseeable future,” he said.
One of the challenges to this growing demand will be bridging the skill gap as Kristen highlighted.
“The momentum that I have seen is like in the era of…pre OpenAI is trying to bridge the skill gap by enabling more people to do SQL. Managing all the infrastructure, models and everything we’ve talked about with LLMs, I feel like that’s deeper in the stack…it’s not more SQL analysts but a different persona.”
An exciting time for data teams
As our expert panel made clear, generative AI is going to have a big impact on how organizations think about and extract value from their data…it just might take a bit longer than those Twitter X posts would have you believe.
It’s an exciting time to be in the data space and have the opportunity to solve some of these important challenges.
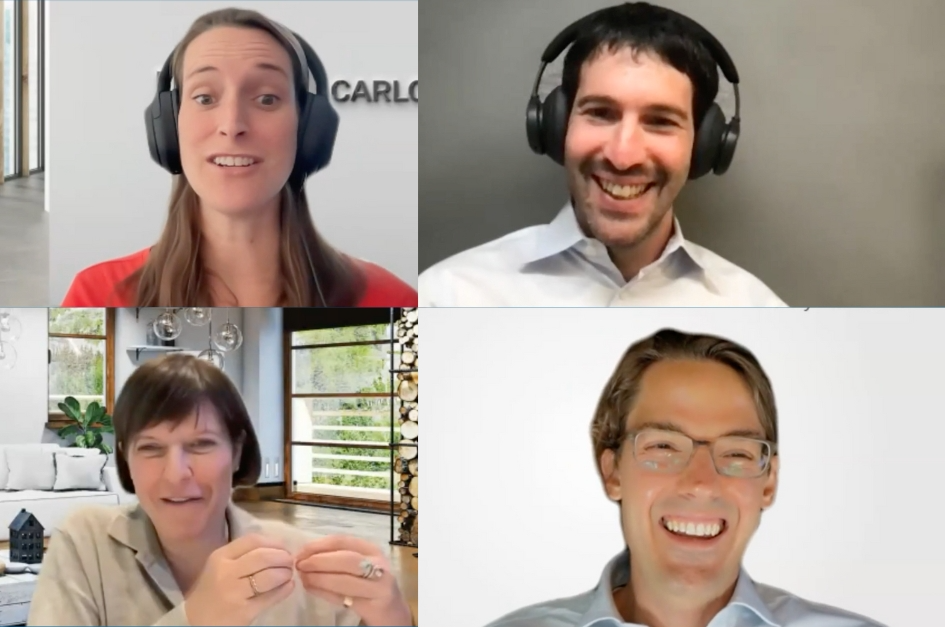
Be sure to watch our panelists exchange views and access the full presentation on-demand.
Interested in how data observability can help with your generative AI strategy? Book a time to talk to us by filling out the form below.
Our promise: we will show you the product.