4 Ways Automation Helps Data Engineering Teams
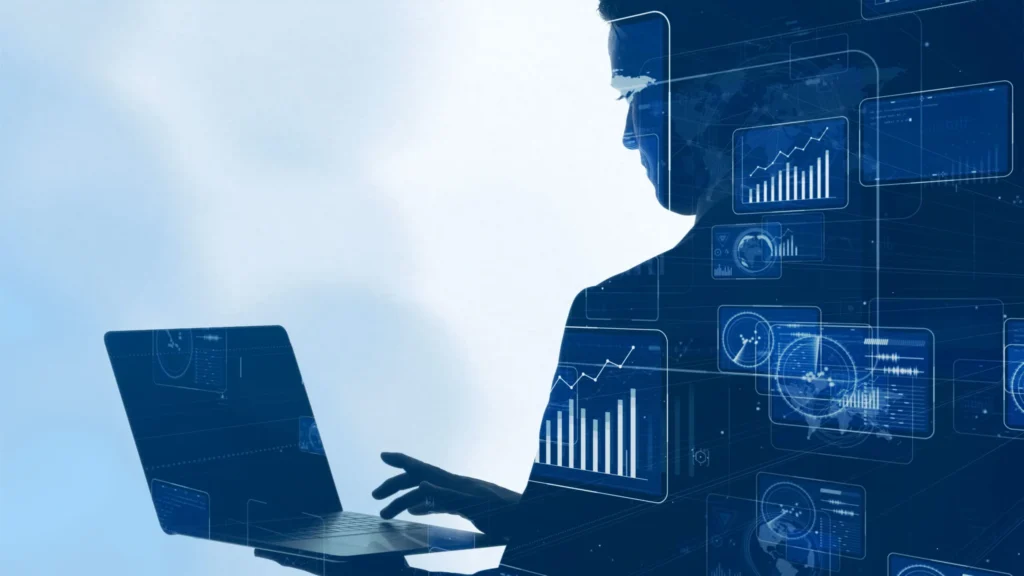
This is a guest post from our friends over at Satori Cyber.
Data-driven organizations generate, collect, and store vast amounts of data. To effectively manage and analyze this data, data engineering teams must navigate a wide range of challenges, including data access, security, compliance, and data observability.
Automation is a missing link in many organizations’ efforts toward data operationalization. As data volumes increase and IT ecosystems are more complex, relying on a manual process to manage data is impractical and difficult to scale. Manual processes are cumbersome and subject to human error.
In this article, we will explore how automation can help data engineering teams overcome these challenges and provide a more seamless and effective data management process.
In this article:
Data Access
Data access is critical for accelerating time-to-value for data-driven organizations. The burden of granting and revoking access to data typically falls to the data engineering teams.
Every time a user wants access to data this needs to be approved by the data engineering team. However, to appropriately grant access to the data, data engineers need to know why the users need access to the data and when this access should be revoked.
This manual data access process can lead to a bottleneck in the data pipeline, slowing down the process of accessing and utilizing data for decision-making. Data engineers end up spending 30-50% of their time granting and revoking access, instead of working on their core projects.
By automating data access controls, through self-service access and frictionless access controls, data engineering teams can streamline the process of granting and revoking data access, reducing manual effort and increasing efficiency.
- Self-service data access users gain immediate access to the datasets available in their data portal with security policies dynamically applied for a specified period of time.
- Frictionless, temporary, and just-in-time access ensures users do not have persistent access to data. Instead, they only have access when they need it.
- Automated workflows using approvals from a data portal or through platforms like Slack, Jira, Salesforce and others.
Automation can also ensure that access controls are consistently applied, preventing errors and reducing the risk of data breaches.
Security
Manual security management is resource intensive for data engineering teams, which need to first locate and then classify sensitive data.
Relying on manual processes not only burdens data engineering teams who already have many projects competing for their attention, it also results in security risks. The need to manually scan for sensitive data also means there can be a time lag between scans, which introduces the security risk of unidentified sensitive data.
Utilizing a data permissions scanner and posture manager ensures that data engineers are informed about who has the potential to access data, which helps mitigate this risk.
Automated processes scan all data stores and discover data, including semi-structured data such as JSON on a continual basis. Once identified, the data engineering team can automatically apply security policies on newly discovered sensitive data.
The ability to automate data access and classification allows data engineering teams to set up real-time monitoring and alerts to detect potential security threats before they turn into significant security incidents. Organizations with automation and AI programs contain data breaches faster and save an average of $3.05 million.
Compliance
Compliance requirements can be complex and time-consuming to implement and maintain, particularly in the constantly evolving regulatory landscape.
Automating compliance checks and audits ensures that data engineering teams are up-to-date with the latest regulations and standards without dedicating extensive time and resources. Automated compliance also helps to reduce the risk of non-compliance and the potential for regulatory fines or reputational damage.
Automating and centralizing auditing and monitoring provides a level of visibility and understanding across all data stores. The ability to add contextual detail and metadata to the audit log enables organizations to easily meet compliance and security requirements.
Automating security measures also helps data engineering teams simplify compliance reporting. Data engineering teams can generate detailed audit trails and automated reports, which can be used to demonstrate compliance with regulatory requirements.
Data Observability
Knowing and understanding the availability and quality of data is important for data engineers. Automating data quality with data observability enables data engineering teams to monitor the health and performance of their systems in real-time and quickly identify and resolve issues.
By automating data quality, data engineering teams can ensure their systems are performing optimally and meeting service level agreements (SLAs). The real-time visibility into the performance of data pipelines, allow data engineering teams to quickly identify and troubleshoot issues.
Automating data quality with data observability also allows data engineering teams to proactively address issues before they become major problems. Automated monitoring can detect anomalies and alert data engineers to potential issues, allowing them to address problems before they impact downstream systems. This can save time and resources by reducing the need for reactive problem-solving and downtime.
Automated data observability platforms like Monte Carlo provide end-to-end data observability, which significantly reduces data engineering resources allocated and data downtime.
Conquer your data before it conquers you
Automating various aspects of data governance can greatly benefit data engineering teams, enabling them to more efficiently and effectively manage their data at scale.
As data volumes continue to grow and IT ecosystems become more complex, it is increasingly important for organizations to embrace automation and data governance best practices to stay competitive in the data-driven landscape.
Interested in how data observability can help you automate your data quality monitoring and accelerate issue resolution? Talk to us!
Our promise: we will show you the product.