How To Implement Data Mesh: Top Tips From 4 Data Leaders
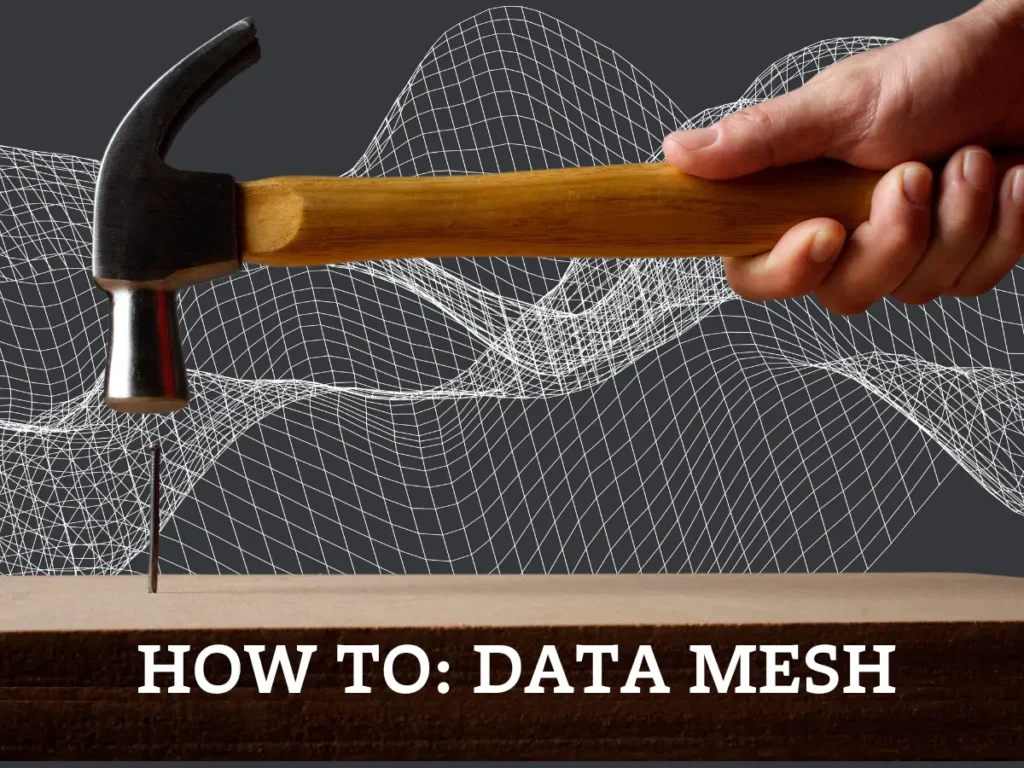
Data leaders across industries are embracing data mesh. It’s easy to be skeptical based on past trends that have come and gone. Those fads forced us to adjust our strategy, overhaul our tech or re-skill our teams.
But the reason data teams want to better understand how to implement data mesh is because it solves genuine pain-points. Specifically, the problems created by an exhaust-friendly data lake and the all-too-often disconnect between teams – of data producers, data consumers and those in between – that contribute to the value chain of a data product.
In addition, data mesh landed at a time when many of us were advancing a data platform strategy whose purpose was to enable – nay, accelerate – the development of the various enriched datasets, dashboards, analytical apps, algorithms and APIs that we might chose to call a “data product.”
Data mesh was the clear and logical framework that articulated this future state. But with a lack of case studies outlining how to implement data mesh, it can be a challenge to visualize exactly how these data mesh principles come to life at your specific organization.
So, how do you get started on this journey?
During IMPACT 2022: The Data Observability Summit, I had the distinct privilege of chatting with three data pros that have direct experience on how to implement data mesh architectures: Max Schultze at fashion and shoe retailer Zalando, Matheus Espanhol at technology solutions provider BairesDev, and Samia Rahman at biotech startup Seagen.
For a quick refresher of data mesh 101, the architectural and organizational approach is centered around four core principles: domain-oriented data ownership, self-service data infrastructure, data as a product, and federated computational data governance.
Read on for five critical tips shared by Max, Matheus, and Samia during our conversation about what it takes to implement data mesh in practice—without sacrificing data quality or getting lost in the pursuit of perfection.
- Recapping the data mesh
- Tip #1: Choose the right pilot project
- Tip #2: Don’t wait on the perfect platform
- Tip # 3: Define self-service for yourself
- Tip #4: Define domains to thrive independently
- Tip #5: Focus on building trustworthy data products
- How to implement a data mesh step-by-step
- How to implement data mesh at your organization
Recapping the data mesh
Before we jump into the specifics of implementing your first data mesh project, let’s take moment to remember what a data mesh is, what it’s for, and how to set up a data mesh within your organization.
What are the components of a data mesh?
A data mesh is made up of four core principals:
- Domain-oriented data ownership: the management and maintenance of each critical data product is federated among domain owners including responsibility for their own ETL pipelines.
- Self-service data architecture: a system of tooling and process that abstracts technical complexity and allows domains to self-serve their individual data use cases from a central platform that includes the data pipeline engines, storage, and streaming infrastructure.
- Data as a product: the critical data assets including analytical, operational, and customer-facing assets, that will be maintained under the data mesh architecture.
- And federated computational governance: a universal set of standards to facilitate collaboration between domains with shared data.
Each of these four elements combine to facilitate, safe-guard, and provide a sense of direction for your data mesh initiative.
Tip #1: Choose the right pilot project
All of our panelists agreed: when you’re ready to begin this journey, start small with one pilot project on one domain team. Working with one team at first gives you a chance to learn valuable lessons for how to implement data mesh that will be essential as you adopt the architecture across your organization over time.
“You need to start with a single team because you don’t know from day one how to do it perfectly,” said Max. “There are no quick wins. You need to go through the learning process to understand what data mesh means in and of itself, and what it means specifically for your organization. You’ll learn that one step at a time and the people you built the first data product with early on will later become your biggest ambassadors to spread the knowledge across other teams.”
For the pilot project, focus on a data product that has clear, quantifiable business value. There’s no point selecting a data product that has unclear demand or value. However, don’t get too ambitious either. It’s probably a good idea to avoid a pilot that overhauls critical financial reporting. You will also want to ensure the domain team you partner with is equipped with the right skills to build and support a data product from day one.
“When we started to develop our data platform, we already had domain-oriented ownership in place,” said Matheus, “but as we thought about treating data as a product, we wanted to prioritize what would be a good solution for data producers and consumers. For example, not all the domains had the roles needed to implement the full self-service concept at first. And we need to be patient with that and make sure they aren’t relying on the data platform team instead of finding their own autonomy.”
Be ready for pushback. More than likely, you are not just altering the way your team works, but how you expect every domain leader and their teams to be accountable for the data they produce, enrich and consume. Expect to regularly communicate the strategy and the value implementing data mesh unlocks for the business, whether that be in terms of speed, scale or revenue.
“The first time we mentioned the concept of data mesh, there was a lot of pushback,” said Max. “Slowly but surely, people began to understand it’s not about buzzwords or hype around the data mesh term—it’s about the concepts that create value for the company. And that’s when it got easier to have a good conversation with more people, up to the point that the major pillars of the data mesh made it into strategic outlooks.”
Tip #2: Don’t wait on the perfect platform
As you implement your pilot project and plan for wider adoption of data mesh, be ambitious but reasonable.
Samia encourages data teams to be realistic and define KPIs they can achieve. “Mistakes I’ve made with past teams include over-indexing on perfect self-service or trying to establish a lead time of delivering a data product within a day. That’s the mission or the vision, but we need to be pragmatic.”
And Max doesn’t recommend trying to overhaul the entire tech stack at once, especially if you’re working in a more established organization.
“Data mesh always describes this beautiful greenfield self-serve setup for data platform and infrastructure,” he said. “But that’s rarely where any company is starting. You usually have 10-15 years of legacy systems of data warehouses, governance, and functions. You have to understand how to evolve your tech step-by-step into the self-service direction to make it scalable.”
Think of how to implement data mesh as though you’re remodeling a house while you’re living in it. Instead of demolishing the existing structure and starting from the ground up, you want to go room-by-room and update your data architecture incrementally, highlighting the golden pathways for domain teams to follow.
Tip # 3: Define self-service for yourself
“So far, I have not seen any two data meshes that look alike,” said Max. “It really depends on your specific organization and the capabilities you need.”
Defining what domain-oriented architecture and self-service data infrastructure look like in your organization all depend on your business needs. For Samia, that means asking questions about real needs versus idealized best-case scenarios.
“Do I need to have self-service in the way I provision a warehouse?” she said. “Or do I really need self-service in the way we do data quality checks? I need to know which reusable capabilities I can make that will give us the most bang for the buck. Because the other things can be manual. Depending on your landscape, just enough is good enough.’
For example, Matheus’s team started to provide self-service by helping data producers ingest data through Fivetran. At Samia’s company, giving domains control over who could access data and simplifying data visualization standards was the top priority.
Tip #4: Define domains to thrive independently
When it comes to defining the domain teams themselves, Max advises that data leaders look at similarities in the way those teams use data in pursuit of their (independent) objectives.
“In e-commerce, for example, you start with everything that relates to the web experience,” he said. “Then you have logistics, and then everything around payments. You have very different parts of the business that are rather obvious in specific business contexts that belong together in one domain.”
Typically, there will remain some cross-domain or shared-domain data that will continue to be managed centrally, often within the data platform team, serving enterprise use cases that cut across two or more domains.
Once you have those domains determined, staff the domain teams with the relevant cross-functional talent and domain expertise to thrive on their own.
“If you bring the really smart domain people and the tech people as close as you can, they will do magic,” said Samia. “We have similar decentralized patterns where we have a data product manager by a domain—finance, commercial, whichever—and they have a cross-functional team with a report developer and a data engineer. Embedding the reporting team with the business team gets you to value a lot faster, because they’re building out their domain data analytics portfolio with the stakeholder in an organic way.”
Tip #5: Focus on building trustworthy data products
Early on teams must wrestle with rules-of-the-road for building data products and the associated governance of the system. These questions can include:
- What are the essential attributes of a data product?
- What standards and policies are enforced globally across domains?
- What decisions are made locally within domains?
Typically, I’ve seen data organizations favor clear standards over heavy governance frameworks, with an emphasis on trustworthy and discoverable data products.
For Matheus, this meant emphasizing trust. “In the beginning, you can’t enforce too many rules without impacting adoption and the learning curve for the domain teams,” he said. “But without product thinking, you end up having one or several data swamps. So we looked at trust as a must-have attribute and use data observability for data producers and consumers to make sure our data is reliable and trustworthy.”
Max shared a view on trust that extended beyond the reliability of the data, ensuring you are addressing business needs.
“You have to understand and build back from what the business needs,” said Max. “This is where I often see people stumbling. They focus so much on how to build a product and lose sight of what they’re building it for. But when you start with the business need and build to that, it becomes much easier to measure, display, and showcase the right requirements — which creates trustworthiness.”
For Samia, this means answering the why before you start to build products. And this can feel unnatural to some technical teams.
“If you come from data engineering, you want to jump into the how,” she said. “But data product teams adhere to ‘answering the why’. We have a templatized set of questions that help us move away from service tickets to human interaction during our intake process.”
Regardless, setting clear standards and policies early on will help to drive consistency and interoperability across data products.
How to implement a data mesh step-by-step
There’s no one-size-fits-all solution for a data mesh architecture. As a theory that relies on a variety of tools and processes to bring about its purposes, a data mesh will look different at every organization. However, there are a few key steps to any data mesh project that can help to point you in the right direction.
- Step 1. Define why your organization would benefit from a data mesh. As exciting as the latest trend might seem, it doesn’t always make sense for every organization. If your data team is too small or you have limited data needs, a data mesh might not make the most sense right away. So start by defining what you hope to achieve from a data mesh and if that’s something you can benefit from now, or if it’s something better left for the future.
- Step 2. Get buy in. The primary conceit of the data mesh is that it federates ownership and responsibility. But that only works if you have buy-in and broad support from the teams and stakeholders being impacted or federated to. So, take your time explaining the project and its benefits, and make sure the broader stakeholders are informed, consulted, and comfortable before moving forward.
- Step 3. Define your data products. Domain differentiation will often depend on the data products in question, so start by defining the data products and who’s using them. If you have five domain teams, but only three critical data products, you might not need to bring every domain into the data mesh project.
- Step 4. Choose 1-2 data products to test. Don’t boil the whole ocean in your first go. Choose 1-2 important but low-risk data products to test with a data mesh architecture. These would ideally be data products that have well documented pipelines, controlled data sources, and a clearly defined domain.
- Step 5. Train your domain teams. Recall that a data mesh is just as much process as it is tooling. So, just because you understand what’s expected and how to do it doesn’t mean your domain leaders will. So take the time to train early and often, and hold regular office hours to give domain leaders and practitioners the time to ask questions and get comfortable. You’ll be relying on these teams to carry the torch on their own in the future, so don’t skimp on the training today.
How to implement data mesh at your organization
As our panelists made clear, the answer to how to implement data mesh will look different at every company. You can learn best practices and get inspired by looking at what other teams are doing, but your data mesh will be as unique as your organization.
-shane murray
We’ve helped teams across industries build trustworthy data products through end-to-end data observability.
Contact us today to talk about your business needs, and how to implement data mesh principles.
Our promise: we will show you the product.