Our Top 5 Data Mesh Articles In 2022
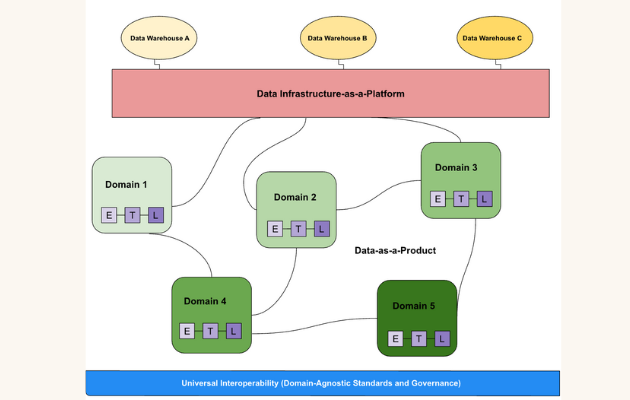
Data mesh is a complex socio-technological data engineering concept, but it doesn’t change too much. The four principles are still the four principles, there are still three experience planes, and automation is still as vital as ever.
This is a good thing! Data mesh is one of those rare transformative concepts that emerged relatively fully formed as a result of creator Zhamak Dhegani’s years of consulting experience captured in a comprehensive 384 page book.
If you are looking for the most complete, definitive guide to data mesh that is the resource for you. However, while the end state remains clear, what is a bit more murky are the practical steps data teams can take to get to that final destination.
To that end, our top data mesh blog posts in 2022 mainly examined real-world implementations and the adaptations data teams made on their journey to data mesh.
Table of Contents
- How We’re Implementing a Data Mesh at Sanne Group
- How To Implement Data Mesh: Top Tips From 4 Data Leaders
- Organizing Talent: Return of the Data Center of Excellence
- How BlaBlaCar Leveraged Monte Carlo For Their Data Mesh Transition
- 4 Data Mesh Principles To Get One Step Closer To Data Nirvana
- The common thread: the data mesh journey begins with a single step
How We’re Implementing a Data Mesh at Sanne Group
This article by Martin French, group head of data engineering at Sanne Group, is a forward looking account of his game plan for transitioning his department from a centralized to decentralized model.
It’s a massive task, and it cannot be done overnight, but Martin explains his plan for maintaining optionality and how the nuances of the business will lead to modifications in how he implements and emphasizes the four pillars of data mesh.
How To Implement Data Mesh: Top Tips From 4 Data Leaders
During IMPACT 2022: The Data Observability Summit, we hosted an in-depth conversation with three data pros that have direct experience on how to implement data mesh architectures: Max Schultze at fashion and shoe retailer Zalando, Matheus Espanhol at technology solutions provider BairesDev, and Samia Rahman at biotech startup Seagen.
Their advice? Choose the right pilot project, don’t wait on the perfect platform, define self-service for yourself, define domains to thrive independently, and focus on building trustworthy data products.
Organizing Talent: Return of the Data Center of Excellence
Our CEO Barr Moses referenced this post from our field CTO, Shane Murray, when she predicted that “data gets meshier but central platform teams remain.”
This article examines the overall trend toward embedded, decentralized data teams and the advantage of maintaining a center of excellence. Shane argues these teams often get the best of both worlds: the agility of decentralized teams and the efficiencies of centralized teams.
How BlaBlaCar Leveraged Monte Carlo For Their Data Mesh Transition
This case study tells the story of why data mesh is so popular among data engineering teams. BlaBlaCar had speed and scale issues that they originally tried to solve by throwing bodies at the problem.
When this didn’t work, they decided to implement data mesh with Monte Carlo helping them to understand how to identify and distribute ownership of key data assets across domains. Today, they have successfully transformed their data engineering organization and improved their data quality.
4 Data Mesh Principles To Get One Step Closer To Data Nirvana
As we previously mentioned, data mesh is a complex concept. At its heart are the four principles (domain-driven data ownership and architecture; data as product, self-service data platform, federated governance) which we describe as simply as we possibly can. This is a great article for those looking for a straightforward introduction to data mesh.
The common thread: the data mesh journey begins with a single step
To paraphrase a Chinese proverb, “the data mesh journey of a thousand miles begins with a single step.”
Where you start, what you emphasize, and the team you first work with will have a big impact on whether your data mesh journey is successful. However, it’s better to get started and adapt then wait until you can launch the perfect data mesh architecture.
Interested in hearing more about data observability, data products and data mesh? Schedule a time to talk to us using the form below.
Our promise: we will show you the product.