How to Create a Data Quality Strategy (in 5 Steps)
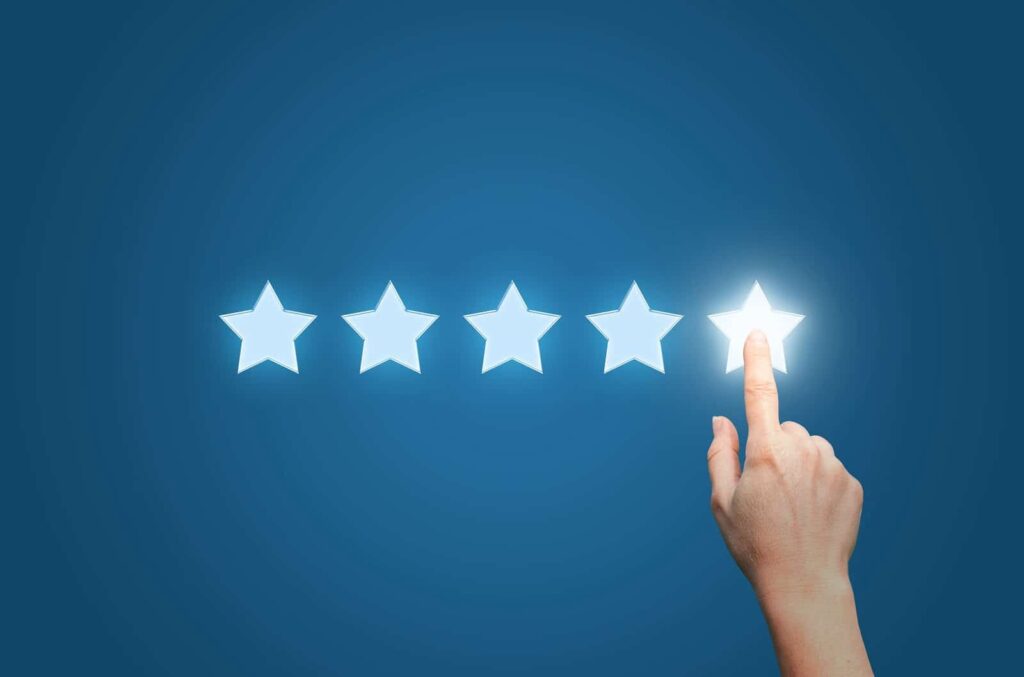
In the last decade, we’ve figured out how to track, collect, store, and query data, but we haven’t mastered our ability to figure out how to make sure that your data can actually be trusted and used.
Dror Engel, Head of Product, Data Services, at eBay, and Barr Moses, CEO and co-founder of Monte Carlo, share five essential steps for establishing a successful data quality strategy.
Recently, a Chief Data Officer (CDO) at a leading financial services company told us that his data organization processed and stored over hundreds of thousands of jobs (up to one terabyte of data) per day.
Despite the scale and speed of his infrastructure, however, he still hadn’t cracked the code on what it took to ensure that this data was actually valuable and trustworthy. Bad data was costing his team millions of dollars per year and firefighting data issues were taking several hours per week of his data team’s time.
Sound familiar?
You’re not alone. According to Gartner, data quality issues cost companies $14 million per year on average, while Forrester calculates that data quality issues take up around 40 percent of a data analyst’s time. Another data leader we spoke with said that bad data accounted for 1,200 cumulative hours per week for her 500-person team.
Fortunately, there’s hope. Over the past few years, the best data teams have started giving data quality and reliability the diligence it deserves. Here’s what we will cover in this post:
- Getting leadership & stakeholder buy-in for your data quality strategy
- Set data quality SLAs
- Spearhead a data stewardship program
- Automate your lineage and data governance tooling
- Create a communications plan for your data quality strategy
- Key takeaways
Getting leadership & stakeholder buy-in for your data quality strategy
Before you start trying to secure leadership and stakeholder buy-in, it’s important to be transparent about the current state of your data quality strategy. Consider how you might answer the following questions:
- How do you measure the data quality of the assets your company collects and stores?
- What are the key data quality KPIs or overall goals you’re going to hold your data quality strategy accountable for meeting?
- Do you have cross-functional involvement from leadership and data users in other parts of the company?
- Who at the company will be held accountable for meeting your strategy’s KPIs and goals?
- What checks and balances do you have to ensure KPIs are measured correctly and goals can be met?
In the same way that having visibility into your data pipelines makes it easy to ensure high data quality, transparency into both your data quality strategy and its incremental progress will be critical when it comes to keeping everyone informed and accountable.
Set data quality SLAs
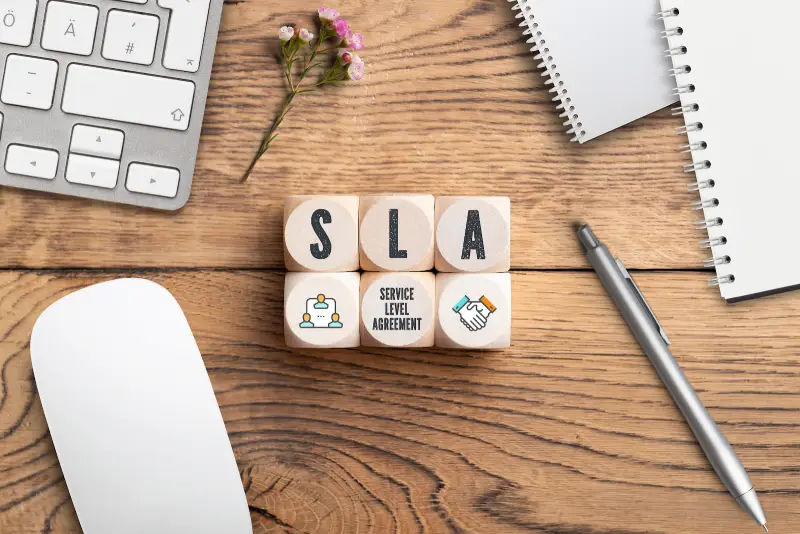
In the same way that software engineering teams set service-level agreements (SLAs) for uptime and downtime, data teams must take a similar approach to setting guidelines around data quality. Defining clear SLAs off the bat will also help with stakeholder buy-in.
Before you tackle your SLAs, it’s critical to understand and align on each part of the data life cycle and how data brings value to your company. The outcome of each phase in this process will determine your corresponding data quality SLAs and measurements. For instance, raw data ingested by your data lake or warehouse need to fulfill different requirements than transformed data rendered in a data warehouse.
Avoid focusing on data quality measurements. Instead, keep it simple. Measure for tangible data quality metrics like completeness, freshness, accuracy, consistency, and validity as opposed to obscure “accuracy” scores or other homegrown measurements. These types of frameworks will only lead to confusion down the road as SLAs shift to meet company priorities.
Spearhead a data stewardship program
If a data quality program launches but no one else at the company hears about it (including leadership), will it have an impact? Probably not. To make sure that data users across the company are aware of why data quality matters, we suggest developing a program for data quality champions to carry the torch and shepherd others through data access, use, and storage best practices.
Make participation and evangelism easy and accessible. Be sure to communicate how data quality affects their functional areas, from marketing to sales, and make it easy for them to share and enforce with their team. Focus on short-term or quick wins to get traction while promoting and executing on the long-term data quality strategy.
Automate your lineage and data governance tooling
With increasingly stringent compliance measures around data access and applications, a manual approach to data quality monitoring as a vehicle for data governance is not cutting it.
Not only is manual data quality monitoring tedious and time-consuming, but these tools can’t keep pace with the speed of innovation across the rest of the data stack (think: ML-enabled data modeling, speedy analytics dashboards, and the data mesh).
Instead, we suggest investing in automated tools that can quickly validate, monitor and alert for data quality issues as they arise. Add the ability to set custom rules, and these technologies have the potential to truly unlock the potential of data for your organization.
Create a communications plan for your data quality strategy
Now that all of the pieces are in place, the final step is to put together a robust and comprehensive, program-level communications plan that will keep leadership in the loop, stakeholders aligned with your project’s progress, and data stewards abreast of their marching orders.
A good communication plan will be bi-directional and keep all involved in the loop on the status of relevant deliverables. A great communication plan will instill confidence in even skeptical parties that your team is in command of the situation, regardless of how far you are from your goals.
Key takeaways
At the end of the day, the goal of your data quality management strategy will be to ensure that teams across the entire company feel empowered to use data that is trustworthy. In fact, we believe a robust and comprehensive data quality strategy makes all the difference when it comes to doing just about anything in data, from scaling an effective data team to building a great data platform.
So pat yourself on the back: by reading this article, you’re already a step (or should we say, five steps) ahead.
Interested in learning more about how to implement a successful data quality strategy? Reach out to Dror Engel or Barr Moses.
This article was originally published in Forbes.
Our promise: we will show you the product.