Data Governance Frameworks: 5 Lessons from McKinsey, Microsoft & More
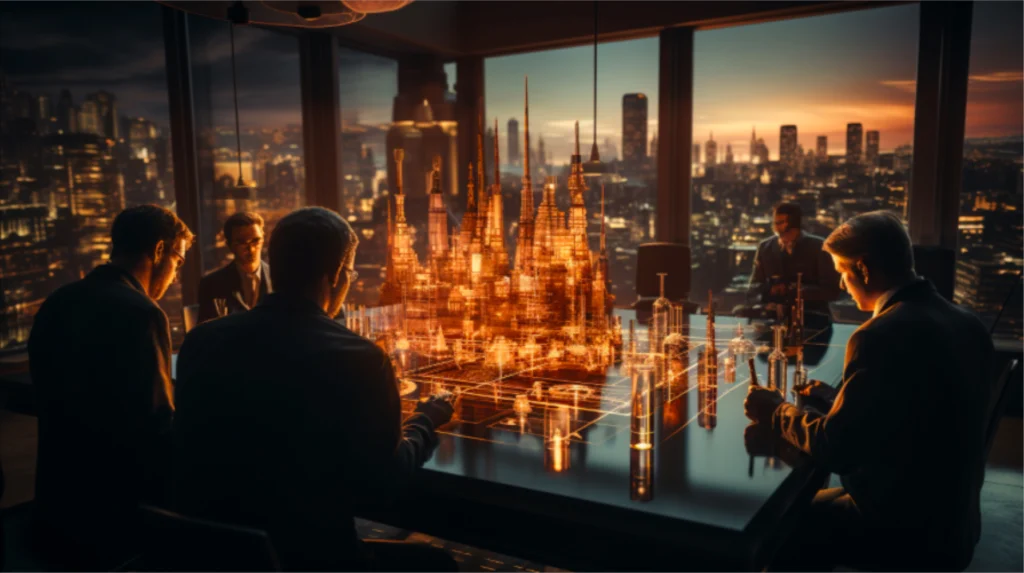
If there’s one thing that’s become abundantly clear in the world of tech, it’s that “data is the new oil.” And, much like oil, data can be a tricky substance – it’s incredibly valuable when refined and put to use, but it’s a flammable mess if not properly managed. That’s where data governance frameworks come into play, acting as the safety protocols and refinery blueprints of the data world.
Data governance frameworks provide a systematic approach for organizations to manage, use, and protect their data.
And who better to learn from than the titans of industry like McKinsey, Microsoft, and Amazon? These trailblazers have laid the groundwork, battled the challenges, and reaped the rewards of effective data governance, and now we’re going to dissect those experiences to bring you six key lessons in data governance framework best practices.
McKinsey: Leadership is central to effective data governance
In their designing data governance that delivers value insight piece, McKinsey’s framework highlights the need for buy-in from business leadership, particularly the C-suite. Once leaders grasp the value of data governance, they become its champions.
They suggest linking data governance efforts directly to ongoing transformation initiatives that already have the attention of senior leadership and assigning each leader several data domains.
Within their domains, leaders then select representatives to act as data-domain owners and stewards to work in sprints to identify priority data based on the value they could deliver, checking in with the senior leadership team every few weeks. Tracking metrics like the costs associated with poor data quality helps ensure the attention and continuing support of top management.
Microsoft: Embed data governance in the design phase
Microsoft ensures that data governance is an integral part of the everyday rhythm of the business, rather than an afterthought, by integrating controls into the engineering DNA of the company.
This method, often referred to as “governance by design,” involves creating a clear framework of data standards and embedding them into the application development process. This framework comprises both internal standards – how data is ingested, processed, accessed, and eventually disposed of – as well as external standards set by government agencies, industry associations, and other relevant third parties.
Some examples include data privacy measures, data retention periods, access controls, and audit trails. Detecting data compliance gaps in these areas before they get published into production reduces the risk of data breaches or non-compliance with regulations.
Amazon: Build a communication plan
Effective communication is key, says Amazon. The retail juggernaut suggests building a communication plan for informing the organization about the data governance standards and policies. The framework of this plan should:
- Highlight the benefits so employees can see how data governance will help them make better decisions in their jobs
- Communicate the rules and policies. This could be included as part of annual training and given to educate new hires
- Provide an escalation matrix, in other words who to contact when there are data issues or security events
Deloitte: It’s not just compliance, there’s opportunity too
While compliance is a key aspect of data governance, the benefits of implementing an effective data governance framework extend far beyond compliance. Deloitte emphasizes advantages like improved decision making, operational efficiency, data quality, risk management, and reputation.
Instead of policies and procedures implemented in response to regulations or after data breaches or other incidents, what they call a “defensive mindset,” they encourage companies to also take an “opportunity mindset,” where data governance is seen as a strategic tool for enhancing business value. For example, addressing data governance issues can lead to more accurate analytics and better decision-making.
SAS: Culture is a critical factor
A culture that values data as a strategic asset is more likely to succeed in implementing data governance, says SAS. Managing resistance, clear communication, celebrating success, and continuous education about data governance are essential to fostering a positive culture.
Incentives and rewards can encourage desired behaviors, reinforcing its importance and motivating employees to contribute. The most effective incentives will depend on your culture, but some examples within this framework include public recognition, career advancement, monetary rewards, certificates or badges, and competitions.
Good data governance begins with good data
As we’ve gleaned from the experiences of these five industry leaders, a robust data governance framework requires leadership support, cultural buy-in, and iterative adaptation. It should be embedded early in the design phase and viewed not just as a compliance tool, but also a strategic asset for creating business value.
But even more important: good data governance begins with good data. Just as the oil industry employs cutting-edge technologies and meticulous inspection to guarantee the quality of their crude, so too should businesses employ data observability tools like Monte Carlo to ensure their data’s quality.
Even the most robust governance can’t make up for flawed raw material. In essence, the oil you extract sets the stage for everything that follows.
Request a demo by filling out the form below and put Monte Carlo to work in your data fields.
Our promise: we will show you the product.