You’re Not Realizing the Full Value of Your Company’s Data
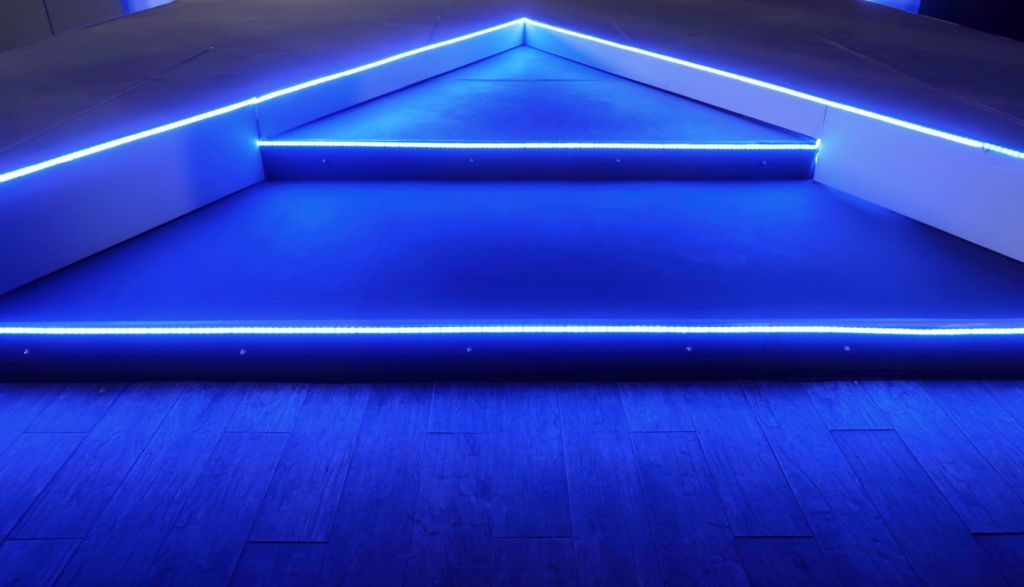
You’re on Snowflake and Looker? Great. But for most companies, having a cloud data stack is just the tip of the iceberg when it comes to operationalizing their data and analytics at scale. We share five non-obvious roadblocks businesses face when becoming data driven and what some of the industry’s leading data engineering and analytics teams are doing to overcome them.
Today, you’ll be hard-pressed to find a company that doesn’t want to be seen as “data-driven” or “data-first.” Yet for most, data analytics is just a buzzword, not an innovative source of critical business value.
In order for your business’s data analytics strategy to reach its full potential, you have to build a culture of data appreciation and adoption across the entire organization. We share five of the most common challenges that stand in the way of analytics excellence—and how you can tackle them.
Challenge #1: Too many pilots, too few scaled initiatives
Many times, data is used to solve siloed problems within the context of pilots and experiments, not fully productionalized projects. This is common, especially in the early stages of building a data-driven enterprise, but it’s a landmine you should address sooner rather than later.
Too many pilots create a misperception in the rest of the company—that data is for experiments, not creating business value. This perception makes it difficult to start building buy-in and a shared understanding of the possible value of data and analytics.
As swiftly as possible, move to a strategic position by aligning your data and analytics vision with your overall business strategy. Identify priorities where data and analytics solutions can explicitly deliver on the most critical elements—and work to unlock trapped value by quickly moving proven proofs-of-concept pilots into production.
As you identify key priorities, ask yourself — what is the goal of your data and analytics solutions? What business questions are you trying to answer? And what actions will you empower business leaders to take based on your work? What value these initiatives will create?
Challenge #2: Organizational bottlenecks
Even if you have well-structured data in place, you need to have the right people with the right skill sets on the right teams to make use of it. The same team that develops a brilliant idea for how to leverage data to solve a problem may not be equipped to test and scale up the solution, creating bottlenecks and eroding confidence in data-backed initiatives.
One proven approach to address this challenge is to adopt a “Hub-and-Spoke” model, with a dedicated hub owning the strategy and governance, talent management, and providing a companywide set of data and analytics services for the entire organization to leverage.
“Hub” accelerates business value by delivering critical services with the right capabilities to the enterprise. “Spoke” teams work on functional roadmaps and execute functional data and analytics initiatives as they have deep functional knowledge, function-specific skill set and understand functional needs and processes better. “Spokes” follow common guidelines and principles set by the “hub”, and are accountable for adoption and value realization of cross-functional enterprise wide initiatives, championed by “Hub.” You should also form agile delivery squads, a cross-functional team of teams with its own defined goal, which they work towards autonomously. Each squad has a ‘product owner’ and prioritize work to be done.
Take a step back and examine your organizational structure. Do you have the right mix of talent with the right tools and access to take an idea to a proof-of-concept to full production? Do you have clearly defined roles and responsibilities and ways of working? Are teams function as One Team, in One Voice, and towards One Goal?
Challenge #3: Lots of data but little insight
When companies try to improve their ROI on analytics, the data itself is rarely the challenge—it’s the ability for employees to glean meaningful insights from a large volume of data. Teams need to know what data is used for which purpose, where they can find it, and how they can access it. We call this data democratization.
To address this challenge, set two major end goals employees should have a single source of truth for data, and the organization should treat data as a strategic, valuable asset that shouldn’t be misused or wasted.
This is not a simple task. The team of teams need to work across several cross-functional teams to get data out of siloed ownership by implementing a common data acquisition framework, and introducing a standard data governance program. You should also implement a proven data catalog system, which enables employees to find and access datasets shared across the entire organization, and to trust that it has reliable data to use in their daily work.
Big accomplishments don’t happen overnight, but leaders can start building momentum by hosting informational sessions or ‘lunch and learns’ that show your teams how to leverage the data and analytics they can access right now in their day-to-day jobs.
As you move towards data democratization, ask yourself: Do you have a clearly defined data strategy? How can you communicate your strategy, and build an appetite within your entire organization to adopt a shared approach to data and analytics?
Challenge #4: Trying to be everything for everyone
Machine learning and other AI disciplines have emerged as powerful solutions for driving profitable growth while generating and scaling analytics. However, as many companies rush to adopt ML and AI, data and analytics leaders face the challenge of trying to be everything for everyone—applying new technologies and methodologies simply because you could, without stopping to determine if you should.
Similar to the first challenge, as you start to introduce AI into your data program, begin by identifying the problems where machine learning and other automated solutions can be most effective and drive meaningful business outcomes.
For example, data quality management, a necessary component of any serious data strategy, often requires significant manual threshold setting and metadata entry. ML-first approaches, like end-to-end data observability, allows data engineers and analysts to focus on projects that actually move the needle, as opposed to ad hoc firefighting or manual toil. With data teams spending 80 percent of their time and companies wasting $15 million annually on data quality issues, data observability enables teams to increase data accuracy with little effort as you apply data across the entire organization.
ML is also used to make predictions, such as how Uber directs its drivers to an area about to surge in demand or how Airbnb recommends prices to maximize revenue in dynamic markets. In every case, the quality of the predictions are entirely dependent on the quality of the data used to train the machine learning models.
If you plan to build custom models, first make sure you have enough data to do the project justice—and be aware of the potential biases that will likely come into play.
Answer the following before leveraging AI and ML: Are our datasets clean enough and accurate enough to remove manual oversight? Have we identified the points where biases may come into play, and do we know how to detect and correct them? Again, this is where a robust approach to data observability can help.
Challenge #5: Security, privacy, and governance
As always, security and privacy are ever-present factors that will impact the success of your data program. As you work to break down silos and increase access to data, you need to make sure that data—including every access point and pipeline it touches—is secure.
Getting the right data governance approach in place early on, with your long-term goals and outcomes in mind, will help you implement and scale analytics more easily over time. You should ensure your entire data stack, including warehouses, catalogs, and BI platforms, are compliant with data governance guidelines for your company, industry and region.
All of these challenges are significant, but not insurmountable. With these best practices, a strategic vision, and the right technology, your data analytics program can drive meaningful change across every business unit and be a force multiplier for years to come.
Implementing a data governance strategy at your company? Reach out to Barr Moses and the Monte Carlo team to learn how we can help.