Improve Data Consistency With Monte Carlo’s Cross-Database Rules
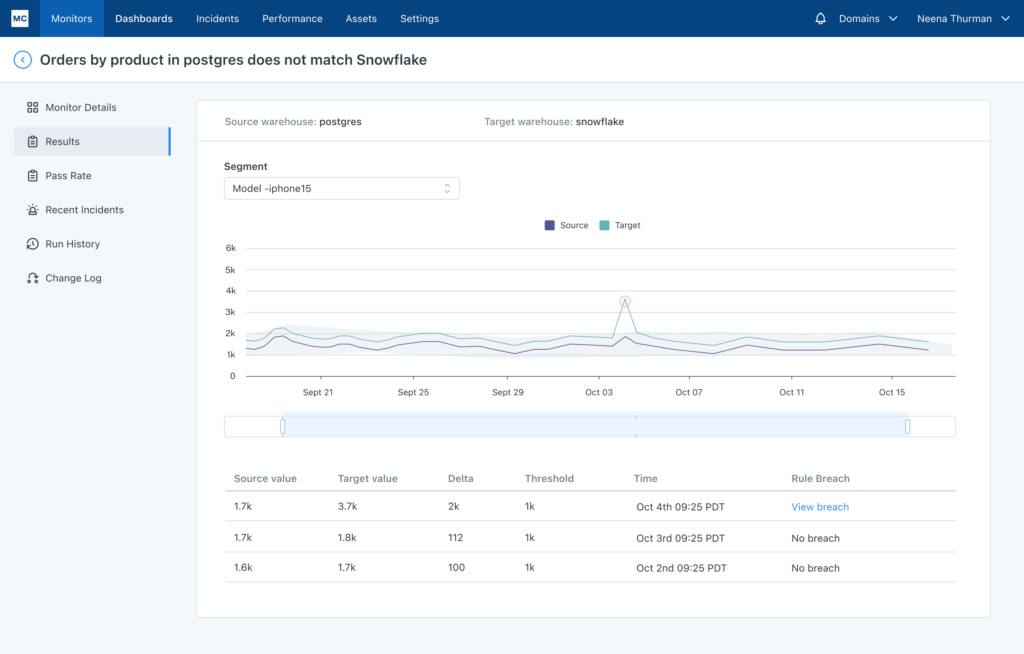
Not only are there a lot of ways data downtime can strike, but there are a lot of places it can strike too.
One of the increasingly common infiltration points is when data is being synced across databases. This typically occurs when data teams want to move data from a transactional, on-premise, or staging database into the raw layer of their analytical data warehouse, lake or lakehouse.
Rather than receiving a direct copy, an error occurs somewhere within the source-to-target sync, and the databases begin to drift apart. This could be the result of an orchestration issue where data was delayed, a schema change in the transactional database that didn’t align with a hardcoded pipeline, or one of many other possibilities.
Now there are now multiple claims to the truth that must be reconciled. Data consistency suffers as multiple values for the same metric begin to propagate across the organization.
That’s frustrating for anyone who has been in a meeting where more time was spent arguing over the data rather than operational action items, but imagine how frustrating it is for an external customer. Two different departments of the same organization might have two different versions of their transaction history!
Monitor data consistency with Comparison Rules
Now, organizations can better monitor and improve their data consistency with Monte Carlo’s Comparison Rules, which makes it easy to compare data across multiple warehouses. Here’s how it works.
Users write two SQL queries – one pointed at the source data warehouse and one at the target – and set an alert threshold for the allowable difference.
An example use case could be if you want to “check that the sum of sales from yesterday is within .1% between source and target.” This can even be done by segment. (If you wanted to check the sum of sales by product, for example).
Comparison Rules work alongside our machine learning monitors that detect freshness, volume, schema, and data quality anomalies as part of one unified data observability solution.
This new capability, along with Monte Carlo’s expanded support for transactional and enterprise data warehouses, provides increased visibility into data reliability further upstream than ever before.
Data consistency builds data trust
Once bad data worms its way into your data platform, it can quickly spread across your interconnected pipelines and tables. Now, you can detect and resolve these incidents before they irreparably damage operations, revenue, and trust.
Want to see how Monte Carlo can help improve your data reliability and data consistency? Set up a time to talk to us using the form below!
Our promise: we will show you the product.